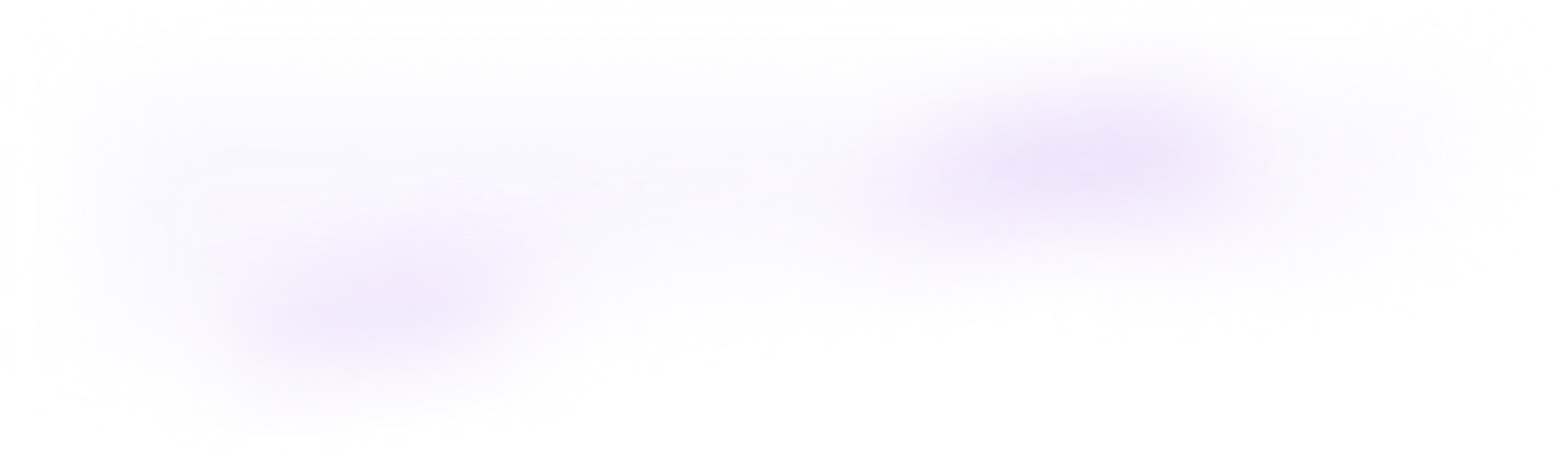
Agentive AI in 2025: Definition, Use Cases, & Best Practices
Discover the power of Agentive AI, its advantages over traditional AI, and how it's transforming industries in 2025.
March 26, 2025
Author(s)
Key Takeaways
Agentive AI is revolutionizing industries by anticipating user needs and making autonomous decisions in real-time.
With its ability to continuously learn and adapt, Agentive AI enhances efficiency and improves user experiences.
Businesses leveraging Agentive AI are driving smarter automation, boosting productivity, and creating more personalized digital interactions.
Agentive AI represents a transformative shift in the realm of artificial intelligence, introducing systems capable of autonomous action and decision-making. Unlike traditional AI, which operates based on predefined rules and requires human intervention for complex tasks, agentive AI systems proactively interact with their environment to achieve specific goals. This autonomy allows them to adapt to new situations, learn from experiences, and perform tasks without continuous human oversight.
The evolution of agentive AI can be traced back to the early developments in AI, where the focus was primarily on creating systems that could process information and provide outputs based on set algorithms. Over time, advancements in machine learning and computational power paved the way for more sophisticated models capable of not just processing data but also making informed decisions. This progression has led to the emergence of AI agents that can autonomously navigate complex environments, a concept that has gained significant traction in recent years.
In this article, we delve deeper into the nuances of agentive AI, exploring its distinguishing features, historical development, and the impact it has on various industries. We will also examine how platforms like Orq.ai are facilitating the development and deployment of these intelligent systems, and discuss the ethical considerations that come with their integration into our daily lives.
Agentive AI vs. Traditional AI
Artificial intelligence has evolved significantly over the years, giving rise to increasingly sophisticated systems. Traditional AI and agentive AI represent two distinct approaches to leveraging machine intelligence, each with its own strengths and limitations. Understanding these differences is crucial for organizations seeking to implement AI-driven solutions that enhance efficiency, personalization, and automation.
Core Differences Between Traditional AI and Agentive AI
Traditional AI: Rule-Based and Reactive
Traditional AI operates within predefined boundaries, relying on structured algorithms, rule-based decision-making, and historical data to generate outputs. These systems excel at pattern recognition and predictive analytics but remain reactive rather than proactive.

Credits: Cubet Tech
For instance, most customer support chatbots and recommendation engines use traditional AI models. They process user inputs and return predefined responses or suggestions based on programmed logic. However, they lack context awareness and struggle with dynamic problem-solving.
Agentive AI: Adaptive and Autonomous
Agentive AI takes a fundamentally different approach by proactively assisting users and autonomously executing tasks. Rather than waiting for explicit input, these adaptive systems anticipate needs, learn from interactions, and take initiative without requiring continuous human intervention.
A prime example of agentive AI is a Copilot that assists software engineers. Instead of merely suggesting code snippets based on previous inputs, it analyzes project requirements, recommends optimal solutions, and even autonomously executes repetitive coding tasks.
Key capabilities that distinguish agentive AI from traditional AI include:
Autonomous decision-making: Making informed choices without human prompts.
Context awareness: Understanding the environment and user intent for more relevant actions.
Tool integration: Seamlessly interacting with multiple applications to complete complex workflows.
Advantages of Agentive AI
1. Enhanced User Experience Through Proactive Assistance
One of the most compelling benefits of agentive AI is its ability to deliver proactive assistance. Instead of merely responding to queries, these systems anticipate user needs and act accordingly.
For example, an agent hub designed for customer support could preemptively surface solutions from a knowledge base, reducing response times and enhancing customer satisfaction. By leveraging Retrieval-Augmented Generation (RAG), these systems pull relevant insights from vast datasets, ensuring more accurate and contextually relevant responses.
2. Increased Efficiency and Productivity
Through complex workflow automation, agentive AI significantly enhances operational efficiency. Businesses that integrate these intelligent systems into their processes benefit from reduced manual effort, faster execution, and improved accuracy.
For industries that rely on client audits and detail testing, agentive AI can streamline processes by autonomously analyzing compliance requirements, generating reports, and flagging discrepancies. Similarly, in software development, AI-driven substantive testing can validate code changes with minimal human oversight, accelerating the deployment cycle.
Key Features of Agentive AI
Agentive AI stands apart from traditional AI by actively engaging with users, making independent decisions, and continuously improving its capabilities. The foundation of this AI-driven transformation lies in four core features: autonomous decision-making, continuous learning, context awareness, and proactive assistance. These attributes enable agent deployment across various industries, from finance and healthcare to customer service and software development.
Autonomous Decision-Making
Unlike conventional AI models that rely on predefined rules and human intervention, agentive AI systems possess autonomous decision-making capabilities. This means they can assess situations, analyze available data, and execute actions without requiring manual input.
For instance, in financial auditing, an agentive AI system with a workpaper feature could autonomously review transaction logs, flag anomalies, and compile reports, reducing the workload for human auditors. Similarly, in e-commerce, an AI-driven recommendation engine can independently adjust product offerings based on real-time user behavior, enhancing human-centric digital experiences without constant oversight.
Continuous Learning and Adaptation
Agentive AI systems improve their performance over time through continuous learning. Unlike static AI models that require frequent retraining, these systems refine their decision-making by processing new data, identifying patterns, and adjusting strategies accordingly.
A prime example of this is RAG (Retrieval-Augmented Generation), a technique that enhances AI models by integrating up-to-date information retrieval. By leveraging custom knowledge repositories, agentive AI ensures its responses remain relevant and precise, adapting dynamically to evolving user needs.
Additionally, AI in data extraction applications can refine its accuracy by learning from past mistakes, reducing errors in document processing, financial reconciliation, and compliance monitoring.
Context Awareness
Traditional AI often struggles to interpret context, leading to generic or irrelevant responses. In contrast, agentive AI excels at context awareness, allowing it to tailor responses and actions based on real-world variables.
Consider an agentive platform deployed in customer service. Instead of providing standard responses, it can analyze the user’s history, emotional tone, and intent to offer a personalized solution. In software development, AI-powered code assistants leverage prompt improvement techniques to refine suggestions based on contextual understanding, ensuring relevance and accuracy.
Proactive Assistance
One of the most transformative aspects of agentive AI is proactive assistance, the ability to predict what a user requires and take action before being explicitly asked. This capability enhances productivity, reduces friction, and optimizes workflows.
For example, an AI-driven AI solution delivery system in project management can preemptively allocate resources, flag potential bottlenecks, and suggest optimizations without requiring manual input. Similarly, in healthcare, an AI assistant can remind patients to take medication, schedule follow-ups, and offer real-time health insights.
Real-World Applications of Agentive AI
Agentive AI is driving intelligent automation across industries, enhancing efficiency, personalization, and decision-making. By leveraging adaptive learning, AI prompts, and proactive assistance, these systems transform how businesses operate and interact with users. Below are key sectors where agentive AI is making a significant impact.
Healthcare
In healthcare, agentive AI is revolutionizing patient care by offering tailored treatment recommendations and real-time health monitoring. Unlike traditional AI systems that rely on static datasets, agentive AI adapts to each patient’s evolving condition, continuously learning and optimizing treatment strategies.
For example, AI-powered virtual health assistants proactively remind patients to take medications, schedule appointments, and even detect early signs of medical conditions through continuous data monitoring. This ability to process unlimited knowledge from medical records, wearable devices, and patient histories allows for precise and timely interventions.
Finance
The financial sector is leveraging agentive AI for fraud detection, risk assessment, and personalized financial planning. By analyzing transaction patterns and detecting anomalies, AI-powered systems can autonomously flag suspicious activities and prevent fraudulent transactions before they occur.
Additionally, AI-driven financial advisors use foundational models trained on extensive market data to provide personalized investment strategies. These systems dynamically adjust recommendations based on economic trends, user spending habits, and risk profiles, ensuring tailored financial guidance without human intervention.
Retail
Retailers are utilizing agentive AI to enhance customer experiences through hyper-personalization and efficient inventory management. AI-powered recommendation engines go beyond traditional suggestion models by anticipating customer preferences and autonomously curating product selections.
For instance, an e-commerce intelligent automation system can analyze browsing behavior, purchase history, and real-time trends to proactively present the most relevant products. On the backend, AI-driven inventory management ensures optimal stock levels by predicting demand fluctuations and automating restocking decisions.
Autonomous Systems
The role of agentive AI in autonomous systems is a subject of debate, particularly in self-driving vehicles. While agentic AI focuses on independent problem-solving and adaptation, agentive AI is more concerned with enhancing human decision-making and performing delegated tasks.
For example, an AI-powered driver assistance system can anticipate traffic patterns, adjust vehicle settings based on user preferences, and suggest optimal routes in real-time. While not fully autonomous, these systems act as AI evolution tools that support human drivers rather than replacing them entirely.
Implementing Agentive AI in Business
For organizations looking to harness the power of agentive AI, a structured approach is essential. Successful implementation requires assessing business needs, integrating AI with existing systems, and measuring performance to ensure a positive return on investment (ROI). With advancements in foundational models and tools like RAG (Retrieval-Augmented Generation), businesses can optimize AI-driven processes for efficiency and adaptability.
Assessment of Business Needs
The first step in adopting agentive AI is identifying processes that would benefit most from AI automation agency solutions. Businesses should evaluate operational pain points, customer service inefficiencies, or data-heavy workflows where AI can enhance decision-making and productivity.
For example, companies with large knowledge repositories can leverage RAG (Retrieval-Augmented Generation) to dynamically retrieve relevant information and provide accurate responses. Similarly, organizations handling vast amounts of customer interactions can deploy AI-powered assistants that offer proactive support, reducing human workload while improving user experience.
Integration with Existing Systems
A critical challenge in implementing agentive AI is integrating it with current infrastructure. AI must work alongside existing enterprise systems, databases, and tools without disrupting workflows.
APIs and Model Compatibility: Businesses can use an AI Gateway to connect agentive AI models with customer service platforms, data management systems, and automation tools. This helps manage API rate limits and handle LLM orchestration.
Incremental Deployment: Instead of full-scale replacement, companies can introduce agentive AI gradually, ensuring a smooth transition.
Workflow Adaptation: Employees should be trained to collaborate with AI, utilizing automation for repetitive tasks while focusing on higher-value work.
By embedding agentive AI into CRM platforms, ERP systems, or customer support channels, organizations can enhance efficiency without overhauling existing processes.
Measuring Performance and ROI
To justify investment in agentive AI, businesses must track its impact using clear performance metrics. Key indicators include:
Operational Efficiency: Reduction in time spent on manual tasks due to AI-powered automation.
Customer Satisfaction: Improvement in response times and accuracy of AI-generated solutions.
Revenue Growth: Increased conversions through AI-driven personalization and decision-making.
By continuously refining AI models and leveraging foundational models for better accuracy, businesses can maximize the value of their agentive AI implementations.
Building Agentive AI: Tools & Platforms
Building effective agentive AI applications requires a suite of development tools that support model integration, testing, deployment, and ongoing optimization. Companies need platforms that can streamline the entire AI development lifecycle while ensuring that AI applications are scalable, adaptable, and secure. Below, we look at some of the key tools available for agentive AI development which offers a practical solution for teams building, shipping, and optimizing AI applications.
Overview of Development Tools
When developing agentive AI, there are several key components to consider:
LLM Integration & Management: Platforms that support integration with multiple large language models (LLMs) enable teams to experiment and select the most appropriate model for their use case.
Prompt Engineering & Optimization: Fine-tuning prompts is crucial for improving the accuracy and responsiveness of AI-driven applications.
Retrieval-Augmented Generation (RAG): By augmenting models with real-time data retrieval, RAG improves the contextual understanding and decision-making ability of agentive AI systems.
Performance Monitoring & Debugging: Observability tools track metrics like cost, latency, and output quality, ensuring the efficient operation of AI models.
Security & Compliance: With the rise of regulations like the EU AI Act, it’s important to choose platforms that offer robust security features to protect sensitive data.
While these components are critical to the development process, businesses benefit from platforms that integrate these features into a single environment, simplifying the process of building and optimizing AI systems.
Orq.ai: Generative AI Collaboration Platform
Orq.ai is a platform designed to help software teams build, ship, and optimize large language model (LLM) applications effectively. With a user-friendly interface and a range of built-in tools, Orq.ai simplifies the development of agentive AI applications while supporting the flexibility needed to meet complex business needs.

Snapshot of common evaluators in Orq.ai's Evaluator Library
Orq.ai offers several features that help streamline the development and deployment of agentive AI applications:
Model Integration: The Generative AI Gateway allows easy integration with over 150 LLMs, enabling businesses to experiment with various models and select the best one for their needs.
Testing & Experimentation: With Playgrounds & Experiments, teams can test different AI models, tweak prompts, and experiment with RAG-as-a-Service to assess AI-generated responses before moving to production.
Performance Evaluation: Orq.ai provides evaluators that assess model performance using feedback mechanisms like RAGAS and human input, ensuring AI outputs meet desired quality standards.
Deployment & Scalability: The platform supports seamless transitions from staging to production, with built-in features like retries and fallback models to ensure dependable deployments.
Operational Insights: Observability & Evaluation tools give teams the ability to monitor metrics like cost, latency, and overall performance to optimize AI application operation.
Compliance & Security: Orq.ai is SOC2-certified and adheres to GDPR and the EU AI Act, ensuring that security and data privacy are maintained throughout the AI development process.
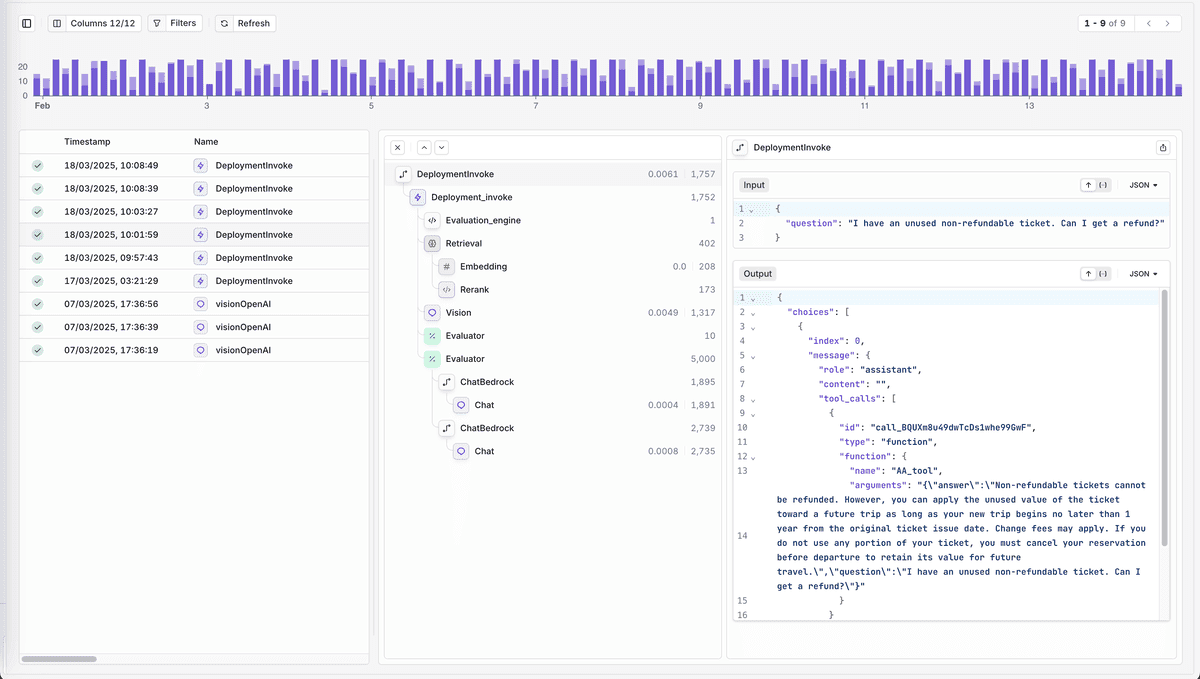
Overview of Traces in Orq.ai's Platform
Book a demo to get an in-depth walkthrough of how our platform supports agentive development workflows.
Agentive AI: Key Takeaways
Agentive AI represents a significant shift in how artificial intelligence interacts with users, moving from reactive systems to more proactive, autonomous solutions. By understanding the core differences between agentive and traditional AI, it's clear that agentive AI offers distinct advantages in terms of efficiency, productivity, and user experience. Its ability to anticipate needs, make decisions autonomously, and continuously learn from data positions it as a powerful tool across various industries.
While the development of agentive AI requires careful planning, from identifying business needs to choosing the right platform for deployment, the right tools and platforms, like Orq.ai, can significantly ease the process. With its comprehensive set of features, Orq.ai helps software teams build, test, and optimize AI applications at scale, enabling businesses to leverage the full potential of agentive AI.
As organizations continue to integrate agentive AI into their operations, it’s essential to keep an eye on both the technological opportunities and ethical considerations. Responsible implementation, ongoing performance evaluation, and a focus on security and privacy will be key to ensuring that AI systems serve both business goals and societal needs.