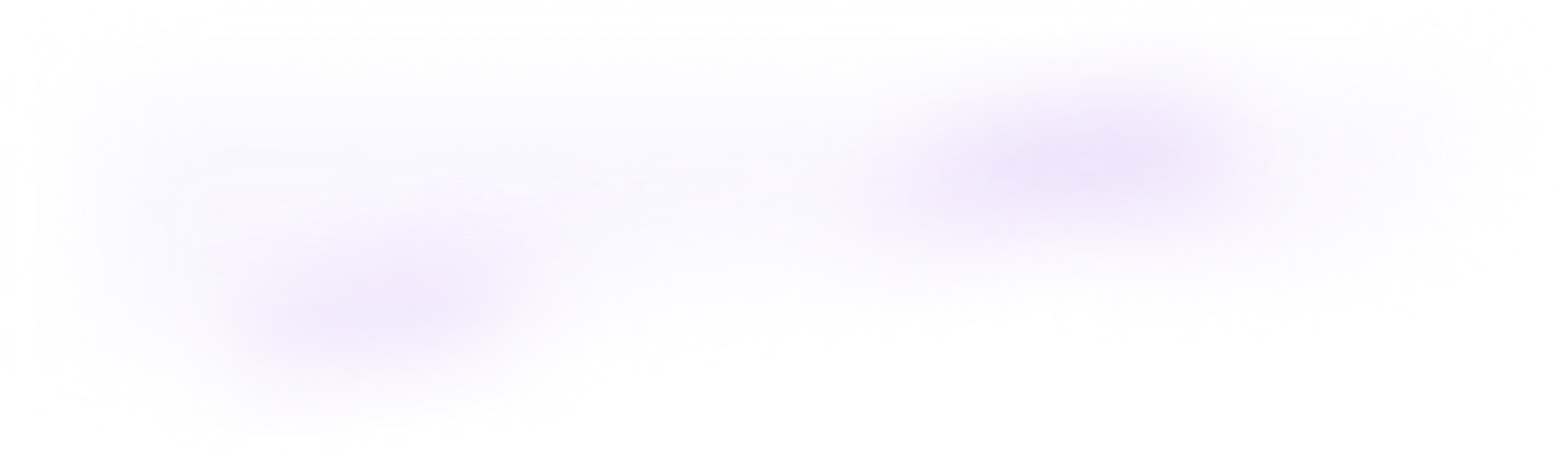
What Purpose do Fairness Measures Serve in AI Product Development? [2025 Guide]
Learn about the importance of fairness measures in AI product development, including key metrics, tools, and strategies for ensuring ethical, unbiased outcomes.
January 6, 2025
Author(s)
Key Takeaways
Fairness measures in AI ensure ethical, unbiased outcomes, building trust and accountability in AI systems.
Key fairness metrics like demographic parity and equal opportunity help guide the development of inclusive AI products.
Emerging tools and strategies, such as real-time monitoring, are revolutionizing how fairness is implemented and evaluated in AI models.
In artificial intelligence, fairness is no longer an optional consideration—it’s a cornerstone of responsible LLM product development practices. Fairness in AI refers to the pursuit of unbiased and inclusive AI algorithms that ensure equitable outcomes across diverse user groups. As AI systems increasingly influence critical sectors like healthcare, finance, and hiring, fairness measures in AI development have become essential for fostering trust, protecting user rights, and ensuring ethical AI product design.
Fairness-aware machine learning not only addresses societal and ethical imperatives but also mitigates the economic risks associated with bias. By incorporating fairness constraints in AI models, organizations can enhance transparency, build user confidence, and mitigate legal and reputational risks when doing product lifecycle management for LLM-based products.
This article explores how fairness measures are transforming AI product development, promoting inclusivity, and aligning with emerging standards for responsible innovation.
Understanding of Bias in AI
Bias in AI arises when systems produce outcomes that disproportionately favor or disadvantage specific groups. These biases can stem from various sources and manifest in different ways, often impacting fairness and reliability. Addressing bias is central to fairness-aware machine learning, as it paves the way for creating ethical and trustworthy AI solutions.
Types of Biases in AI
AI systems can be affected by different types of biases, which can lead to unfair or discriminatory outcomes. These biases arise in various forms and impact decision-making in diverse ways:
Data Bias
Data bias occurs when the training dataset does not represent the diversity of the real world. For example, underrepresentation of certain demographics can lead to skewed results, necessitating the use of unbiased AI training data to mitigate these disparities.Algorithmic Bias
Algorithmic bias arises from design decisions that inadvertently favor specific groups. This is where AI fairness implementation strategies, such as pre-processing or in-processing adjustments, come into play.Interaction Bias
Interaction bias emerges during real-time system use, often influenced by user behavior or system feedback loops. Continuous monitoring and fairness-aware machine learning techniques can help address these issues.
Sources of Bias in AI
Biases in AI are often rooted in different stages of development, from data collection to model deployment. Understanding the sources of bias is essential for addressing them:
Historical Bias
Biases embedded in historical data reflect societal inequities. For instance, hiring algorithms trained on biased data perpetuate systemic inequalities unless corrected using fairness measures in AI development.Systemic Bias
Broader societal and institutional inequities can also seep into AI models. Implementing fairness constraints in AI models ensures that systemic biases are identified and addressed at the design stage.Design Decisions
Choices made during model development, such as feature selection or weight assignments, can unintentionally introduce bias. Ethical AI product design emphasizes proactive bias mitigation in AI systems to reduce these risks.
Impact of Bias on Outcomes and User Trust
Unchecked bias in AI can lead to discriminatory practices, undermine user trust, and damage organizational reputation. By prioritizing fairness measures in AI development, organizations can ensure equitable outcomes while aligning with responsible AI development practices. This proactive approach not only mitigates risks but also fosters societal trust and regulatory compliance.
Role and Purpose of Fairness Measures
Fairness measures are vital for advancing responsible AI development practices. They ensure that AI systems are not only efficient but also equitable, ethical, and aligned with societal values.

Credits: MDPI
By addressing bias and fostering inclusivity, fairness measures serve as a foundational pillar in creating AI products that people can trust and rely upon.
Building Ethical AI and Societal Trust
Fairness measures are essential for mitigating the risks associated with biased AI systems. Ethical considerations in AI products demand that organizations proactively address disparities that could harm marginalized communities. For instance, AI fairness auditing and AI fairness assessment tools help identify potential issues in algorithms before they are deployed. These tools provide actionable insights, ensuring that AI fairness in decision-making systems aligns with societal expectations and regulatory requirements.
When organizations prioritize fairness, they contribute to building societal trust in AI technologies. This trust, in turn, drives user adoption and fosters long-term success by demonstrating a commitment to ethical AI practices. Moreover, robust AI fairness benchmarks provide clear metrics to measure and maintain fairness across industries, setting the stage for consistent innovation in ethical AI.
Ensuring Inclusivity and Equitable Outcomes
AI systems must deliver benefits to all users, irrespective of their demographic background or socioeconomic status. This is where AI equity frameworks come into play, offering structured approaches to promoting fairness and inclusivity. By employing algorithmic fairness metrics, organizations can measure and ensure equitable outcomes for diverse groups.
For example, AI fairness regulations emphasize the importance of unbiased decision-making in sectors like recruitment, lending, and criminal justice. Organizations can integrate AI bias detection methods to preemptively identify and rectify discrepancies in their systems, ensuring that fairness is not an afterthought but a core objective.
Inclusive AI algorithms designed with fairness constraints reduce the likelihood of exclusionary practices, making them pivotal in fostering diversity and inclusivity. These measures also help organizations adhere to AI fairness regulations and avoid reputational damage caused by discriminatory outcomes.
Mitigating Legal and Reputational Risks
Bias in AI is not just an ethical concern—it’s a legal and business risk. With AI fairness impact assessment tools, organizations can evaluate their models for compliance with emerging AI fairness regulations. These assessments are crucial for addressing potential violations that could result in legal challenges or regulatory fines.
Furthermore, fairness measures protect organizations from reputational harm. Public scrutiny of AI systems has increased, and instances of bias can erode trust in a brand’s commitment to ethical practices. By integrating AI discrimination prevention strategies and leveraging AI fairness auditing techniques, organizations can build resilient and trustworthy AI products that align with both societal and legal expectations.
Core Fairness Metrics
Fairness metrics are critical tools for evaluating and ensuring accountability in AI systems. These metrics guide decision-making processes by providing measurable benchmarks to promote equity, prevent discrimination, and foster ethical outcomes in AI models. Understanding and applying these metrics in real-world contexts—such as healthcare, finance, recruitment, and criminal justice—helps organizations meet regulatory standards while mitigating biases.
Definitions of Core Fairness Metrics
Demographic Parity
Demographic parity ensures that AI systems deliver unbiased outcomes by requiring equal treatment across demographic groups. For example, in loan approvals, demographic parity would mean that individuals from different racial or gender groups have equal chances of receiving loans, provided they have similar qualifications. This metric helps minimize biases rooted in systemic discrimination and ensures balanced opportunities for all.Equal Opportunity
Equal opportunity focuses on creating unbiased outcomes by ensuring that individuals in similar circumstances have an equal likelihood of favorable results. This is particularly relevant in areas like hiring practices or AI-based hiring platforms, where gender biases and racial biases can undermine ethical standing. By addressing these issues, equal opportunity metrics support fair and inclusive decision-making processes.Disparate Impact
Disparate impact measures whether certain groups are disproportionately affected by AI-driven decisions, even when those decisions are unintentional. For instance, systemic discrimination in healthcare algorithms can lead to unequal access to treatment for certain racial or socioeconomic groups. Addressing disparate impact helps organizations align their AI models with social justice objectives and ethical outcomes.
Examples and Applications of Fairness Metrics

Credits: Holistic AI
1. Healthcare
In healthcare, fairness metrics are vital for mitigating biases in diagnosis and treatment recommendations. By relying on diverse data sets, organizations can prevent discrimination and ensure that AI models provide equitable healthcare solutions to all patients, regardless of their background.
Finance
Fairness metrics are essential in financial decision-making processes, particularly in areas like loan approvals and credit scoring. For example, organizations must ensure that their algorithms adhere to regulatory standards to avoid perpetuating racial biases or gender biases in lending practices.Recruitment
AI-based hiring platforms are transforming recruitment processes, but they also risk reinforcing systemic discrimination. Incorporating fairness metrics ensures ethical standing by addressing potential biases and promoting balanced opportunities for candidates of all demographics.Criminal Justice
In criminal justice, fairness metrics help mitigate biases in predictive policing and sentencing algorithms. These tools are crucial for achieving unbiased outcomes and fostering public trust in AI-driven systems.
By integrating fairness metrics, organizations can not only promote equity but also enhance their ethical standing, meet regulatory standards, and create AI systems that align with principles of social justice and accountability.
Tools and Processes for Implementing Fairness
Implementing fairness in AI requires a structured approach and the right tools to integrate, measure, and monitor fairness metrics. From defining objectives to evaluating outcomes, the process involves a combination of strategic steps and technical strategies. Tools play a pivotal role in ensuring that fairness is consistently embedded into the AI development lifecycle, enabling teams to build inclusive products and deliver AI solutions that align with ethical standards.
Key Steps in Integrating Fairness
Identifying Objectives
The first step in implementing fairness is to define the specific fairness goals for your AI model. These objectives could range from minimizing gender or racial biases in recruitment algorithms to ensuring equal treatment in credit scoring. Clearly defined objectives ensure that fairness remains a focal point throughout the development process.Selecting Metrics
Once the fairness objectives are set, the next step is selecting the appropriate fairness metrics to measure the model’s performance against these goals. Metrics like demographic parity, equal opportunity, and disparate impact can guide teams in assessing and refining their AI systems. These metrics serve as essential benchmarks to ensure that AI systems deliver unbiased outcomes and meet regulatory standards.Monitoring and Evaluation
Continuous monitoring is essential to maintain fairness in AI models as they evolve. Real-time analytics enable teams to track the model’s performance and detect emerging biases. Regular evaluations—both automated and human-driven—help refine the system over time, ensuring that AI systems remain aligned with fairness objectives.
Technical Strategies for Fairness Implementation
Pre-processing: Pre-processing techniques involve cleaning and transforming data to remove biases before feeding it into the model. This step is crucial for addressing issues like underrepresentation of certain groups or problematic patterns in training datasets. By ensuring the data used in AI models is diverse and unbiased, organizations can prevent discrimination in the final model outputs.
In-processing: In-processing strategies are applied during the model training phase to directly adjust the learning process and ensure fairness constraints are integrated. This can include adjusting model weights or applying algorithms designed to promote equitable outcomes throughout the training process.
Post-processing: Post-processing involves tweaking the model's predictions or outputs to ensure fairness objectives are met. This might involve adjusting thresholds or modifying decision boundaries to correct for any bias that may have emerged during the training phase. This step is often necessary when working with complex AI models that may have inherent biases even after pre-processing and in-processing adjustments.
Orq.ai: LLMOps for Fairness Implementation
Orq.ai offers robust tools to monitor, evaluate, and optimize AI systems in real-time, ensuring that fairness is continuously upheld throughout the AI development lifecycle. Here’s a quick overview of our evaluation and observability capabilities:
Real-Time Monitoring: Track the performance of your AI models in real time, with detailed logs and intuitive dashboards.
Custom Evaluations: Integrate programmatic, human, and custom evaluation methods to assess AI models from multiple angles.
Performance Optimization: Continuously optimize AI performance by leveraging insights from real-time monitoring to refine models.
Transparent Reporting: Access comprehensive reports that highlight any discrepancies or biases, ensuring accountability in decision-making.
By providing these tools, Orq.ai helps AI teams ensure that their models meet fairness benchmarks and maintain ethical standards, offering both transparency and control over model outputs.
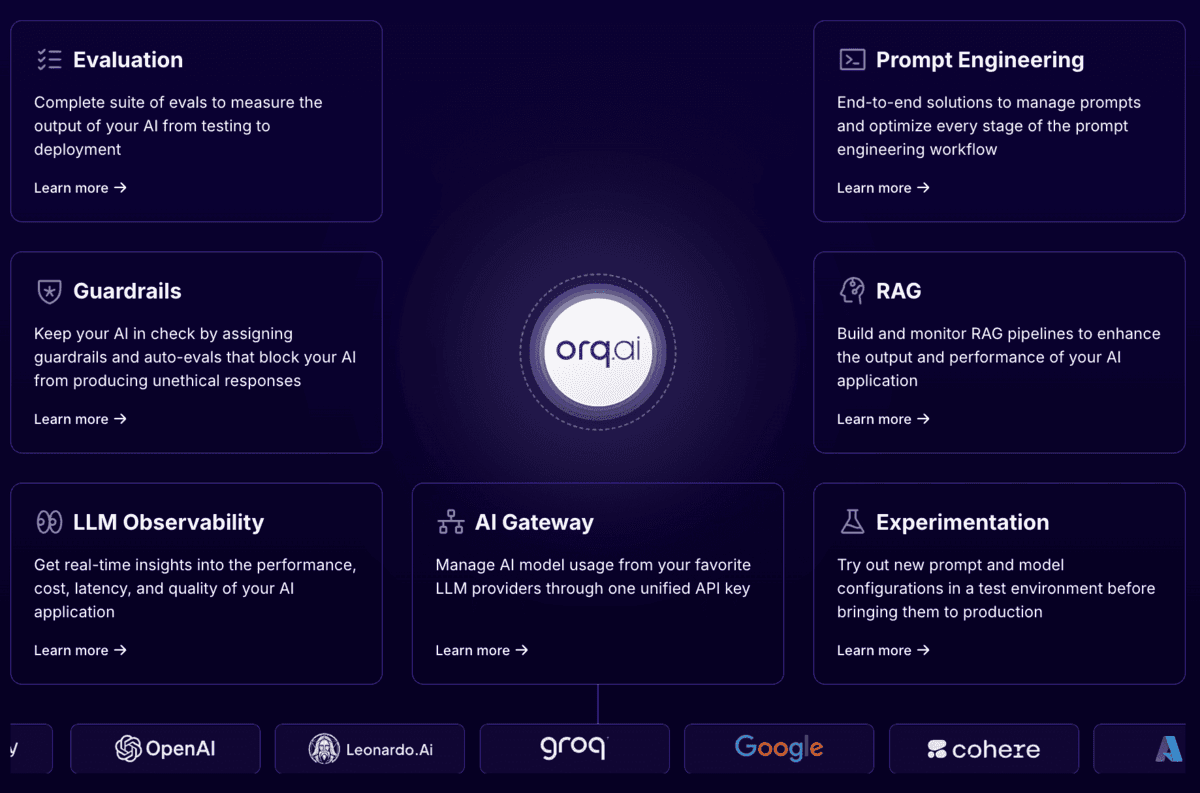
Overview of Orq.ai platform
Book a demo of our platform or visit our technical documentation to leverage our LLMOps platform's observability and evaluation features.
Regulatory, Ethical, and Practical Considerations
As AI technology continues to grow and influence various sectors, compliance with relevant AI regulations and ethical guidelines becomes a vital concern for organizations. Understanding these regulations not only ensures that AI systems are legally compliant but also protects organizations from reputational harm while promoting fairness.
Overview of Relevant AI Regulations and Ethical Guidelines
Several key regulations and ethical frameworks govern AI development:
EU AI Act: This regulation outlines specific requirements for high-risk AI systems, focusing on transparency, accountability, and fairness.
GDPR: The General Data Protection Regulation impacts AI systems by emphasizing data privacy and the protection of personal information.
OECD AI Principles: The Organization for Economic Cooperation and Development has outlined principles to ensure AI promotes human rights and fairness across different sectors.
IEEE Standards: The Institute of Electrical and Electronics Engineers focuses on creating ethical guidelines for AI systems to prevent harm and ensure fairness.
Future of Fairness in AI
The future of AI fairness will be driven by new innovations and deeper integration of inclusivity. As AI continues to grow, advanced tools will be essential for detecting and mitigating biases early in the development process.
Innovations in Bias Detection and Mitigation
Advanced Bias Detection: Emerging algorithms that uncover hidden biases in complex AI models.
Real-Time Audits: Continuous, automated fairness audits to monitor AI models throughout their lifecycle.
Advances in Fairness Evaluation Tools
The next generation of fairness tools will offer more sophisticated methods to evaluate AI, ensuring that fairness metrics are fully aligned with ethical guidelines. These tools will become more customizable, helping teams assess fairness across multiple dimensions and adapt to diverse use cases, such as healthcare, hiring practices, and finance.
Emerging Trends in Inclusive AI Development
Diverse Data Sets: Expanding datasets to better reflect underrepresented populations and reduce bias.
Cross-Disciplinary Collaboration: Bringing together ethicists, sociologists, and AI professionals to design more inclusive algorithms.
Fairness by Design: Moving towards an approach where fairness is a foundational aspect of AI development, not just a post-development correction.
These developments will drive more ethical AI practices and help create systems that are not only effective but also equitable and inclusive.
Fairness in AI development is not just a technical requirement—it's an ethical obligation. As AI continues to shape industries worldwide, implementing robust fairness measures ensures inclusive, unbiased, and transparent outcomes. By adopting the right metrics, tools, and processes, organizations can build AI systems that foster trust, mitigate legal and reputational risks, and adhere to evolving regulations. Moving forward, the integration of fairness by design will be essential to achieving truly responsible AI, empowering teams to create AI solutions that benefit all members of society.