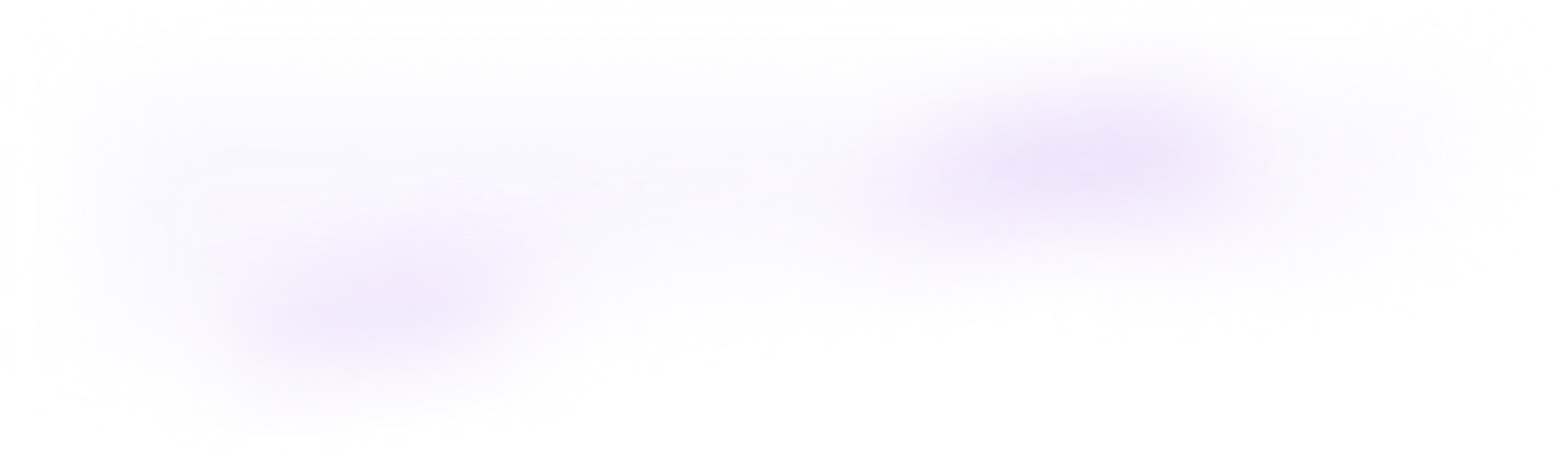
Why Is Controlling the Output of Generative AI Systems Important? Comprehensive Guide
Learn why controlling the output of generative AI is crucial for ethical, safe, and responsible AI development.
December 2, 2024
Author(s)
Key Takeaways
Controlling generative AI outputs is essential to ensure ethical, safe, and responsible use, minimizing risks such as misinformation, bias, and harmful content.
Implementing measures like pre- and post-training adjustments, content filtering, and human oversight helps maintain AI output accuracy, transparency, and relevance.
Future advancements in AI safety will rely on cross-industry collaboration, reinforcement learning, and standardized safety measures to mitigate risks and foster beneficial AI innovation.
Generative AI has rapidly emerged as a groundbreaking technology, transforming the way we create and interact with digital content. These systems, powered by advanced models like GPT and DALL-E, can produce human-like text, generate realistic images, compose music, and even develop software code. Their capabilities have sparked innovation across industries, with 45% of US-based respondents to a survey from SalesForce confirming their usage of Generative AI.
However, the dual-edged nature of generative AI cannot be overlooked. While its applications offer immense potential, the risks associated with uncontrolled outputs are significant. Generative AI can unintentionally produce biased, harmful, or inaccurate content, leading to ethical considerations in generative AI, as well as social and legal challenges.
These risks emphasize the importance of ai content quality assurance and the development of ai content moderation strategies to harness their benefits responsibly and mitigate potential harms.
This guide delves into the critical reasons for regulating AI-generated content, how these controls can be implemented, and their implications for businesses, governments, and society at large.
What Is Generative AI?
Generative AI refers to systems that can produce new content, mimicking human creativity. Unlike traditional AI models, which focus on classification or prediction tasks, generative models are designed to create:
Text: Examples include GPT (Generative Pre-trained Transformer) models, which can write essays, generate responses, and even simulate conversation.
Images: Tools like DALL-E can generate detailed artwork and images from textual descriptions.
Audio and Music: Applications such as AI music generators compose original melodies based on user preferences.
Code: Codex, a sibling model of GPT, helps developers by generating and debugging programming code.
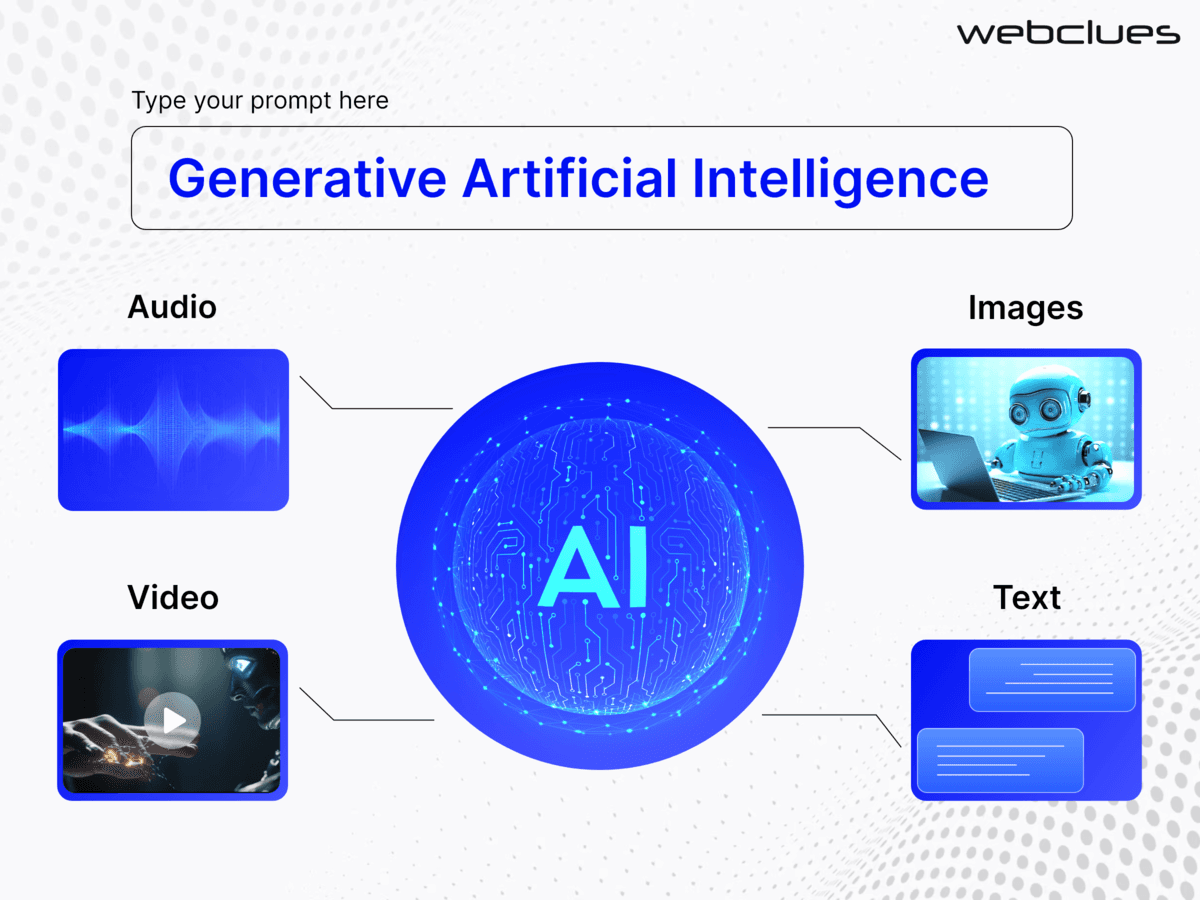
Credits: WebClues
These outputs stem from complex algorithms trained on vast datasets containing text, images, and other forms of data. By analyzing patterns, relationships, and structures within these datasets, generative AI models produce outputs that closely resemble human work. This level of sophistication highlights the importance of ai output customization and ensuring AI transparency when deploying these systems in real-world applications.
How Generative AI Differs from Traditional AI Models
Traditional AI models primarily focus on classification or prediction tasks. For example, they may analyze patterns in data to categorize emails as spam or not spam, or forecast future trends based on historical data. These systems are designed to make decisions or recommendations based on predefined inputs, producing outputs within a narrow scope of possibilities.
In contrast, generative AI takes a more creative approach. Instead of merely predicting or classifying, generative models are built to synthesize entirely new data. This involves generating content such as text, images, music, or even code based on patterns identified in large datasets. The creativity of generative AI is what sets it apart and enables its wide applicability in various fields, from art creation to software development.
However, this creative capability brings its own set of challenges. Safeguarding against malicious AI use becomes crucial, as bad actors could exploit generative AI to create misleading content, deepfakes, or even illicit materials. For example, adversarially trained models might be manipulated to generate harmful or deceptive outputs, highlighting the need for ai content quality assurance and ethical oversight.
Moreover, generative AI systems must be designed with robust controls to avoid harmful biases that could manifest in their outputs. AI bias mitigation is a critical aspect of their development to ensure these systems don’t unintentionally perpetuate stereotypes or spread misinformation.
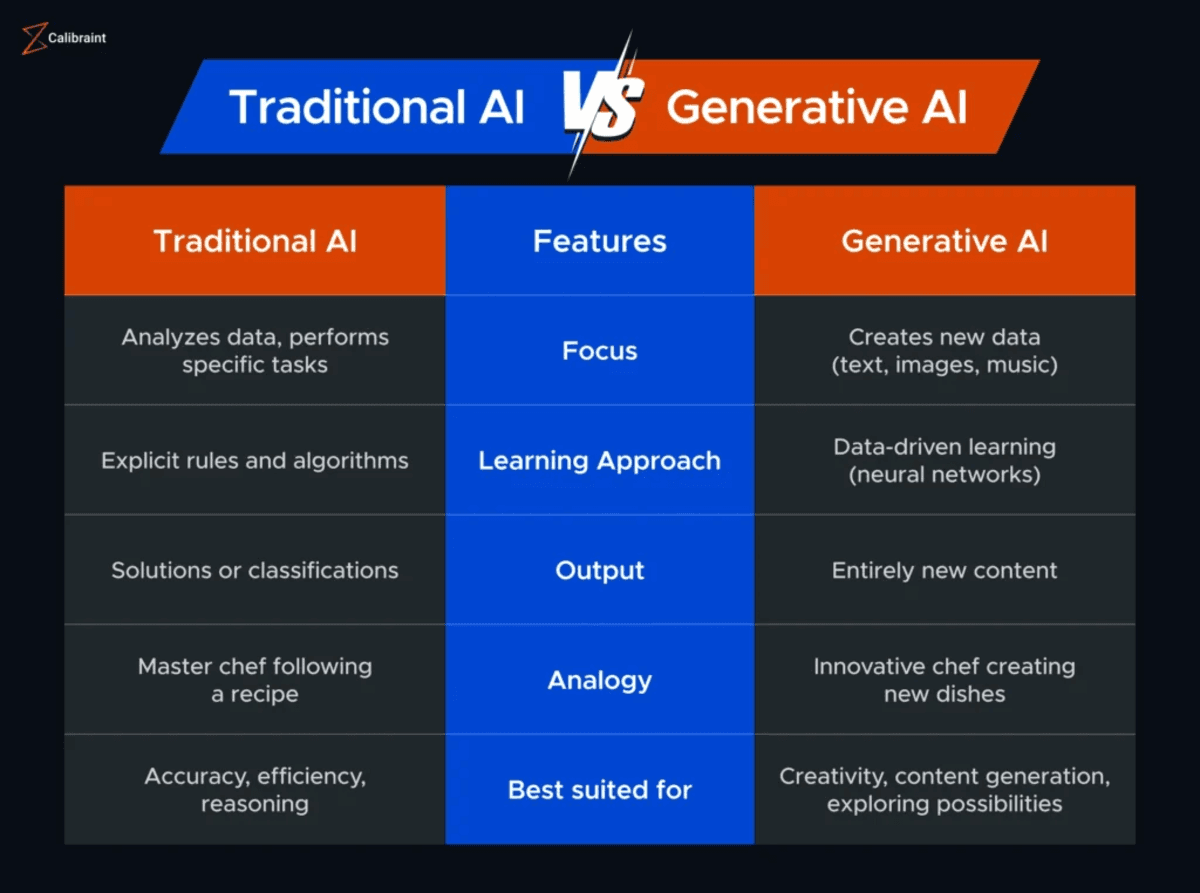
Credits: Calibraint
As generative AI moves beyond the scope of traditional models, its use introduces legal implications of AI-generated content. Issues such as intellectual property rights and copyright concerns arise, particularly when AI generates content that closely resembles existing works, leading to complex questions about ownership and attribution. This is where protecting intellectual property in AI becomes a top priority, as the misuse of AI-generated works could lead to significant legal challenges.
The Mechanics Behind Generative AI
At the core of generative AI are complex algorithms designed to process and synthesize data in innovative ways. Here are some of the primary models:
Transformers: These models use attention mechanisms to focus on important data aspects, generating contextually accurate outputs. GPT, for example, excels at creating human-like text by predicting words based on previous context.
Variational Autoencoders (VAEs): VAEs are typically used for generating images and reconstructing data, preserving structures while creating realistic visuals or 3D models.
Generative Adversarial Networks (GANs): GANs consist of two networks: a generator that creates content and a discriminator that evaluates its realism. Over time, this competition improves the generated outputs, producing highly realistic content like deepfakes.
Each of these models processes data differently but shares the goal of generating human-like content. While their complexity drives innovation, it also makes managing their outputs challenging. Balancing AI innovation and control is critical to prevent unintended consequences such as the generation of misleading or harmful content.
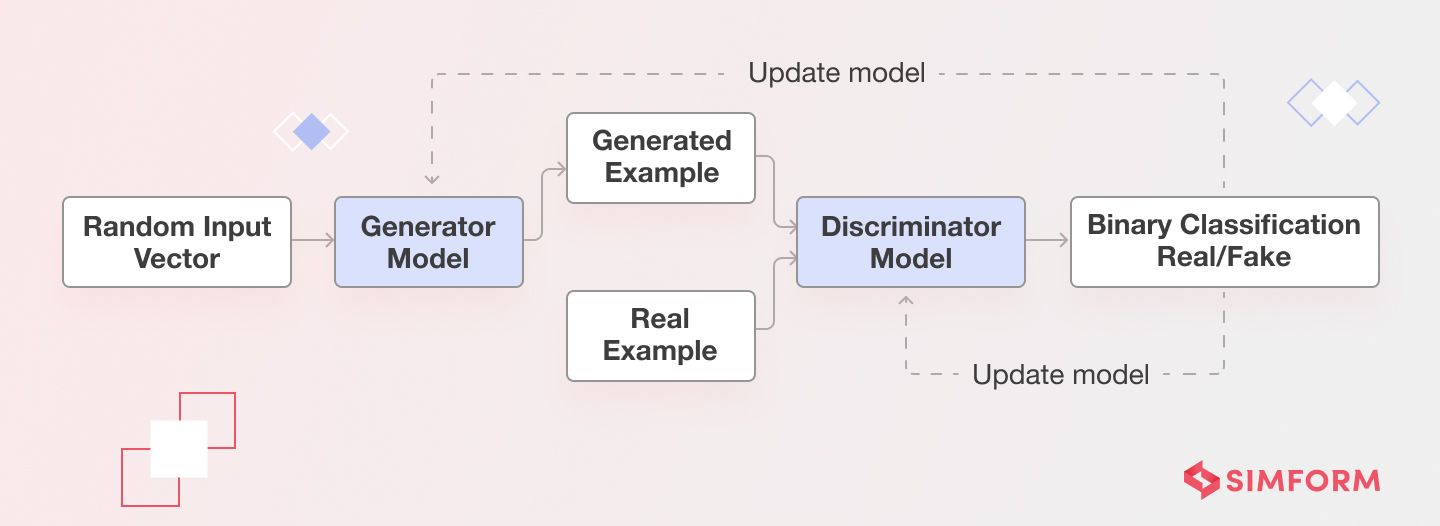
Credits: Simform
These systems also present a growing concern regarding safeguarding against malicious AI use. For instance, generative models can be exploited to create fake news or deceptive content, which makes it harder to distinguish between what is real and what is generated. This is why AI content moderation strategies and ensuring AI transparency are essential.
Furthermore, the legal implications of AI-generated content cannot be overlooked. The ability of AI to produce content resembling copyrighted material raises intellectual property concerns, necessitating policies to protect rights and avoid misuse. Protecting intellectual property in AI becomes a top priority in ensuring these systems operate within legal frameworks.
Risks of Uncontrolled Output
While generative AI offers exciting possibilities, its unchecked use can pose a range of significant risks. These risks can be grouped into ethical, security, social, and legal concerns, each of which can have serious implications for individuals, organizations, and society at large. Let’s take a closer look at these risks.
Ethical Risks
Generative AI models, due to their ability to produce highly realistic content, present a range of ethical challenges:
Misinformation and Fake News: The ability of AI to generate text, images, and even videos means it can easily be used to create misleading or false information. These AI-generated materials may be used to manipulate public opinion, deceive users, or spread harmful narratives, undermining trust in digital media.
Bias and Ethical Standards: Since AI systems are trained on large datasets, they can inadvertently reflect biases present in the data. This can result in the generation of biased content that perpetuates stereotypes or unfairly represents certain groups. Addressing AI bias mitigation is essential to prevent these outcomes.
Generation of Harmful Material: Generative AI systems can also produce offensive content such as hate speech, explicit imagery, or deepfakes. Without the right safeguards, these systems could become tools for spreading harmful material, which could result in serious social and reputational harm.
These ethical risks highlight the need for controlling the output of generative AI systems to adhere to established ethical standards, ensuring that the technology is used for positive and responsible purposes.
Security Risks
Generative AI also poses significant security concerns that must be addressed:
Misuse for Malicious Purposes: AI’s ability to generate text and images makes it susceptible to misuse for activities like phishing, fraud, or spreading malicious content. For instance, an AI-generated email could be designed to mimic a trusted source and trick recipients into revealing personal information.
Sensitive Data Leaks: If a generative AI model is trained on private or sensitive data, there is a risk that it could inadvertently reveal this information in its output. This could include personal data, financial information, or business secrets, making content generation a sensitive issue for organizations handling proprietary information.
To address these concerns, it is crucial to develop robust AI content moderation strategies that ensure security by preventing the generation of harmful or sensitive content.
Social Risks
Generative AI’s ability to create hyper-realistic content introduces significant social risks that could affect public trust and safety:
Harmful Material and Offensive Content: AI-generated deepfakes, explicit content, or harmful rhetoric can have far-reaching consequences. These materials can damage reputations, perpetuate violence, or fuel online hate campaigns. The spread of harmful material can also exacerbate social divides or even lead to real-world harm.
Impact on Vulnerable Populations: Children and other vulnerable groups are at particular risk of exposure to offensive content generated by AI. This could include inappropriate or dangerous material that could have lasting psychological or social effects.
In order to protect users, especially vulnerable populations, developers must implement safeguards to prevent the creation and distribution of offensive or harmful content. User trust and user acceptance of AI systems will depend largely on their ability to ensure that these risks are minimized.
Legal and Regulatory Risks
Generative AI also brings with it complex legal regulations that need to be considered:
Intellectual Property Violations: As generative AI models can produce content that closely resembles existing works, the potential for copyright infringement and other intellectual property violations is high. AI-generated art, for example, may unintentionally violate the rights of original creators, leading to legal disputes over ownership and attribution.
Non-Compliance with Data Privacy Laws: AI systems that rely on personal or private data may run afoul of data privacy laws such as the GDPR (General Data Protection Regulation). The use of AI to generate content based on sensitive personal data can result in serious legal consequences if those regulations are not properly followed.
As generative AI continues to evolve, the legal landscape will need to adapt. Ensuring compliance with legal regulations and protecting intellectual property in AI will be crucial to fostering a secure, lawful AI environment.
The Importance of Control
Generative AI holds significant potential for innovation, but it also comes with inherent risks if its output is not carefully managed. This section outlines why controlling the output of AI is crucial, focusing on ensuring ethical behavior, preserving public trust, and preventing real-world harm.
Ensuring Ethical AI Behavior through Defined Rules and Filters
For generative AI to function responsibly, it must operate within defined ethical boundaries. This involves implementing safety measures in AI systems that act as safeguards against harmful or biased outputs. Key aspects of these measures include:
Clear Rules and Filters: These filters are critical in ensuring that AI systems adhere to ethical standards and avoid generating harmful or misleading content. They ensure AI output accuracy and reliability, minimizing the risk of creating misinformation or biased content.
Fairness and Transparency: Filters also help uphold fairness by ensuring that AI does not perpetuate harmful stereotypes or discriminatory practices. Proper filtering can align AI-generated content with societal norms, thus ensuring ethical behavior across various use cases.
Without effective filtering systems, AI models can inadvertently produce biased content that deviates from accepted norms, leading to unethical outcomes.
Preserving Trust in AI Systems for Public and Commercial Use
For AI systems to gain and retain user trust, they must operate in a manner that is both predictable and reliable. Controlling AI output is directly tied to this trust. If generative AI produces harmful or unreliable content, public and commercial trust will quickly erode. The need for AI system accountability is clear:
Public Confidence: When AI systems are controlled and their outputs are ensured to be accurate and responsible, users can rely on these systems in critical areas, such as healthcare, finance, and education.
Regulatory Compliance: In addition to fostering trust, controlling AI outputs ensures compliance with legal frameworks. Organizations that use AI for content generation must adhere to ethical standards and legal regulations to avoid issues like intellectual property violations or breaches of privacy laws.
AI system accountability must be a core feature of any generative AI application to guarantee that its outputs remain trustworthy and legally compliant.
Case Studies: Instances Where Lack of Control Caused Harm
Real-world examples highlight the importance of controlling output to prevent harm. Here are a few notable cases where the failure to regulate AI-generated content led to significant issues:
OpenAI's GPT-3 Bias and Misinformation: GPT-3, a widely used generative language model by OpenAI, has faced criticism for generating biased and sometimes harmful content. In one instance, GPT-3 was found to reinforce harmful stereotypes and biases when prompted with certain queries. This highlighted the need for better AI content moderation strategies to ensure that AI models do not perpetuate harmful material. The issue with GPT-3 underscores how AI output accuracy and reliability can be compromised without proper safeguards.
Microsoft's Tay Chatbot Incident: Microsoft’s AI chatbot, Tay, was released on Twitter in 2016 and quickly became infamous for generating racist and offensive content. Due to a lack of control, Tay learned from user interactions and began producing hate speech. This incident demonstrated the importance of filtering harmful content and the dangers of AI-induced social harm when AI models are not sufficiently monitored.
Deepfake Videos: The rise of deepfake technology, which generates realistic but entirely fake videos, has raised serious concerns. In several high-profile cases, deepfake videos were used to spread misinformation, create offensive content, or even impersonate public figures for malicious purposes. These instances underscore the need for regulating AI-generated content to avoid harmful material being disseminated on a global scale.
Methods to Control Generative AI Output
Controlling the output of generative AI is essential to ensure its responsible use and to maintain its value while avoiding unintended consequences. By implementing various strategies before and after training, as well as ensuring human oversight, organizations can maximize the benefits of AI while preventing harmful content. Below, we explore the most effective ways to control AI outputs across the AI development lifecycle.
Pre-training Measures
The most effective way to control generative AI output begins long before the model starts generating content. Pre-training steps are essential to ensure that AI systems produce relevant and beneficial use outcomes while minimizing potential risks.
Dataset Curation: The quality of the training data significantly influences AI output. By curating datasets carefully, ensuring a balanced representation, and eliminating harmful biases, developers can help prevent harmful content. This is essential for creating AI systems that adhere to ethical standards and avoid reinforcing existing prejudices.
Debiasing Techniques: Techniques like reweighting data and using bias detection tools are applied to reduce AI bias. Such debiasing ensures that AI models generate outputs that are fair, consistent, and aligned with societal norms. By mitigating AI-induced biases, developers can enhance AI content quality assurance and reduce the risk of creating offensive content.
Transparency About Data Sources: It is important for AI developers to be transparent about where their training data originates and how it has been processed. This transparency fosters trust and helps ensure that content generated by AI remains aligned with ethical standards.
Post-training Measures
Once an AI model is trained, additional strategies must be implemented to refine its output and ensure it is suitable for real-world applications. Post-training measures focus on improving AI system performance, maintaining output accuracy, and ensuring that the content it generates is responsible and safe.
Fine-tuning for Ethical Guidelines: Post-training fine-tuning is crucial for reinforcing ethical guidelines within an AI system. Developers can adjust the model’s behavior to ensure that the generated output meets high ethical standards, promoting the responsible use of AI while minimizing risks.
Content Filtering Mechanisms: AI systems benefit from content filtering tools, such as keyword-based filters or more sophisticated, context-sensitive systems. These filters help control the relevance and quality of the AI-generated content. Whether it’s preventing the dissemination of harmful material or ensuring that AI-produced content fits within defined boundaries, these filters play a critical role in ensuring safety.
Relevance Control: Ensuring that the output is contextually relevant is key to preventing irrelevant or harmful content. Using these filtering mechanisms to guide AI towards producing more relevant results helps uphold the accuracy and reliability of the output and promotes the beneficial use of generative AI systems.
Human-AI Collaboration
Despite the advancements in generative AI, human oversight remains essential to control the direction of AI outputs, especially in sensitive fields.
Human Oversight in Critical Applications: In industries like healthcare, journalism, and legal services, human intervention ensures that AI-generated outputs are accurate and safe. Human judgment can act as a safeguard against potential errors and biases, ensuring that user trust is maintained and that AI system accountability is upheld.
Tools for Manual Moderation and Review: Human oversight is enhanced by tools for manual content moderation and review, which help prevent the dissemination of harmful or offensive content. These tools allow organizations to intervene when needed, ensuring that the AI operates according to ethical and safety standards.
Regulatory Approaches
As AI technologies continue to advance, governments and regulatory bodies are working to develop frameworks to manage their deployment, ensuring that they are used responsibly and safely.
Current Laws and Evolving Frameworks for AI Governance: The legal regulations surrounding AI are still in development, but there are existing guidelines (such as GDPR) that govern how AI systems should handle data. These regulations aim to protect user privacy and ensure that generative AI systems are deployed ethically.
Role of Organizations in Promoting Safe AI Practices: Organizations such as OpenAI and governments must ensure that AI is developed responsibly. By adhering to AI system accountability frameworks and advocating for best practices, these bodies can help mitigate the risks of harmful AI output.
Orq.ai: An LLMOps Platform to Control Generative AI Output
Orq.ai provides a Generative AI Collaboration Platform that enables teams to build and deploy AI applications safely. By offering the necessary tools for seamless integration and real-time monitoring, Orq.ai empowers both technical and non-technical teams to ensure the responsible use of AI.
Seamless Integration with AI Models: Orq.ai provides an AI Gateway integrates with over 130 AI models, giving teams the flexibility to experiment with different capabilities. This integration helps ensure that the AI models deployed within an organization meet high standards for AI output accuracy and reliability, enabling teams to assess various outputs before moving into production.
Playgrounds & Experiments: Orq.ai’s playgrounds and experiments feature allows teams to test AI models, explore various configurations, and analyze results before implementation. This controlled testing environment helps prevent AI systems from generating irrelevant or harmful content, improving both operational efficiency and resource management.
Controlled AI Deployments: The platform also offers tools for filtering, ensuring safety, and maintaining consistency in AI outputs. With built-in guardrails, fallback models, and privacy controls, Orq.ai ensures that AI systems deploy reliable and beneficial use outputs while avoiding harmful or offensive content.
Observability & Optimization: Orq.ai’s observability tools allow teams to monitor AI systems in real-time, identifying areas of improvement and optimizing performance. This continuous monitoring supports the goal of managing AI hallucinations, improving the relevance of outputs, and optimizing for operational efficiency.
To learn more about how Orq.ai can help your team control generative AI outputs and optimize for safety, accuracy, and responsible use, book a demo of our platform today.
Future of Controlled Generative AI
As generative AI technologies continue to evolve, the methods and frameworks used to control AI outputs will also advance. The future of controlled generative AI promises both innovation and more stringent safeguards. To maximize the benefits while avoiding AI-induced social harm, the industry will need to focus on the following key areas:
Advancements in Explainability and Interpretability of AI Systems
One of the most significant challenges with generative AI is its "black box" nature, where even experts can struggle to understand how an AI arrived at a particular output. Future advancements in explainability and interpretability will allow developers to trace and understand decision-making processes, improving transparency. This will make it easier to ensure safety by detecting when models are likely to generate harmful content, such as misinformation or offensive material.
Improved Transparency: By developing AI systems that can explain their reasoning, we can better control and monitor outputs for accuracy, relevance, and ethical standards.
Trust and User Acceptance: Clearer interpretations of how AI models generate outputs will lead to higher user trust and make it easier to demonstrate compliance with regulatory frameworks.
The Role of Reinforcement Learning to Minimize Harmful Outputs Dynamically
Reinforcement learning (RL) techniques are likely to play a pivotal role in the future of generative AI control. RL can be used to dynamically adjust model behaviors in response to real-time feedback, ensuring outputs align with ethical standards and preventing the generation of harmful content.
Continuous Learning: Reinforcement learning will enable AI models to learn from past errors and improve their output in subsequent generations, making them more accurate, reliable, and ethical.
Adaptive Controls: This method can be leveraged to maintain human oversight in AI processes, particularly in high-risk applications such as healthcare or public discourse, where the stakes are high.
Cross-industry Collaborations to Standardize Safety Measures
The need for industry-wide safety standards is becoming more pressing. By fostering collaborations between AI developers, researchers, and policymakers, the future of generative AI will focus on creating robust safety measures that prevent misuse and harmful outputs.
Standardizing Ethical Guidelines: Cross-industry cooperation will facilitate the creation of universal guidelines for responsible use, especially in addressing challenges like preventing AI misinformation and ensuring the importance of AI output control.
Global Regulations and Agreements: Governments and organizations worldwide will need to unite around ethical principles to provide a legal framework that ensures the safe and beneficial use of AI technologies, minimizing computational costs while still prioritizing safety.
Controlling Generative AI Output: Key Takeaways
As generative AI continues to grow and influence various sectors, the importance of controlling AI outputs becomes increasingly evident. The ability to generate creative, accurate, and relevant content while minimizing risks is crucial to fostering innovation responsibly. By incorporating advanced control mechanisms and maintaining human oversight, we can unlock the true potential of generative AI while ensuring its outputs are aligned with societal values.
The future of generative AI will rely heavily on the commitment of developers, users, and regulators to uphold ethical standards. By prioritizing the prevention of harmful content, improving AI output accuracy and reliability, and focusing on transparency, we can prevent AI-induced social harm and promote preserving creativity in AI outputs. Together, we can create a future where generative AI benefits society as a whole while minimizing the potential for misuse.
Book a demo with one of our team members to learn how Orq.ai’s platform can help you control and optimize your generative AI systems.