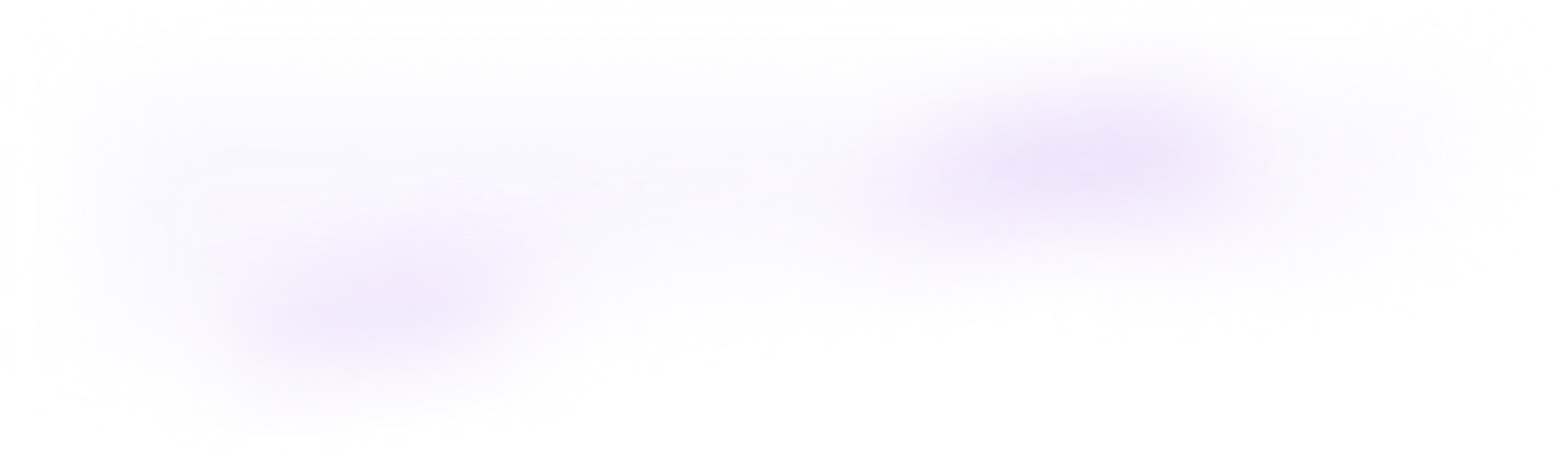
How to Keep Your AI Projects on Track? 5 Best Practices for Managing AI Projects
Discover 5 top practices to keep your AI projects on track. Learn effective strategies to manage timelines, budgets, and teams to ensure the success of your business' transformation.
November 22, 2024
Author(s)
Key Takeaways
Managing AI projects successfully involves addressing unique challenges like data dependency, ongoing updates, and stakeholder collaboration to deliver measurable outcomes.
From maintaining cost control to ensuring data privacy, their insights help organizations navigate the complexities of AI project lifecycles effectively.
By supporting cost tracking, privacy protection, and collaborative workflows, Orq.ai enables teams to manage AI development with greater precision and security.
This article was originally published on Neoteric.eu. Republished with permission.
Using AI isn’t just a trend—it’s a competitive necessity. But here’s the challenge: managing AI projects that align with business goals without getting buried in the technical weeds. Generative AI, in particular, brings enormous potential, but it also requires tight coordination across teams. In these projects, it’s not enough for just the data scientists and engineers to drive the work. You need product teams, developers, and non-technical staff working side by side to ensure the AI is on course to deliver what the business actually needs.
This collaborative approach is called Human-in-the-Loop (HITL). HITL keeps teams involved at key stages of the AI project lifecycle, helping bridge the gap between technical and business goals by having teams continuously monitor, guide, and improve the model’s output. This means that business leaders can stay focused on strategy but with regular checkpoints to ensure that project deliverables stay aligned with this strategy.
Getting these projects right isn’t about mastering algorithms; it’s about mastering cross-functional alignment. In this article, you will read how to prevent your AI and machine learning projects from veering off course, ensuring that technical and business teams remain focused on a common goal from start to finish.
How are AI projects different from traditional software projects?
Before we get into tricks and best practices for managing an AI project, let’s understand how they differ from traditional projects and what specific actions these differences impose.
On the surface, both might seem to follow a similar playbook—define the goals, set the budget, allocate resources, and roll out updates. But AI introduces a unique layer of complexity that requires us to rethink the way we manage projects.
First, AI projects rely heavily on data rather than strictly on code, making outcomes less predictable. This dependency means that managers must prioritize data quality and constantly monitor for bias, which can drastically affect results. Building checkpoints for data validation and testing becomes essential to ensure that models perform as expected.
AI projects also don’t “finish” in the traditional sense; they require ongoing retraining and updates as new data and business needs evolve. Rather than aiming for a one-time launch, you need to plan for an ongoing lifecycle, with regular evaluations and adjustments to keep models relevant and effective.
Finally, the nature of AI requires continuous, close collaboration between technical and business experts to ensure the model aligns with real-world goals. Unlike traditional software projects, where different teams can often work in defined phases, AI projects require ongoing input from both sides to adjust models, interpret outputs, and refine objectives. For project managers, this means actively facilitating cross-functional collaboration throughout the entire project, not just at key milestones.
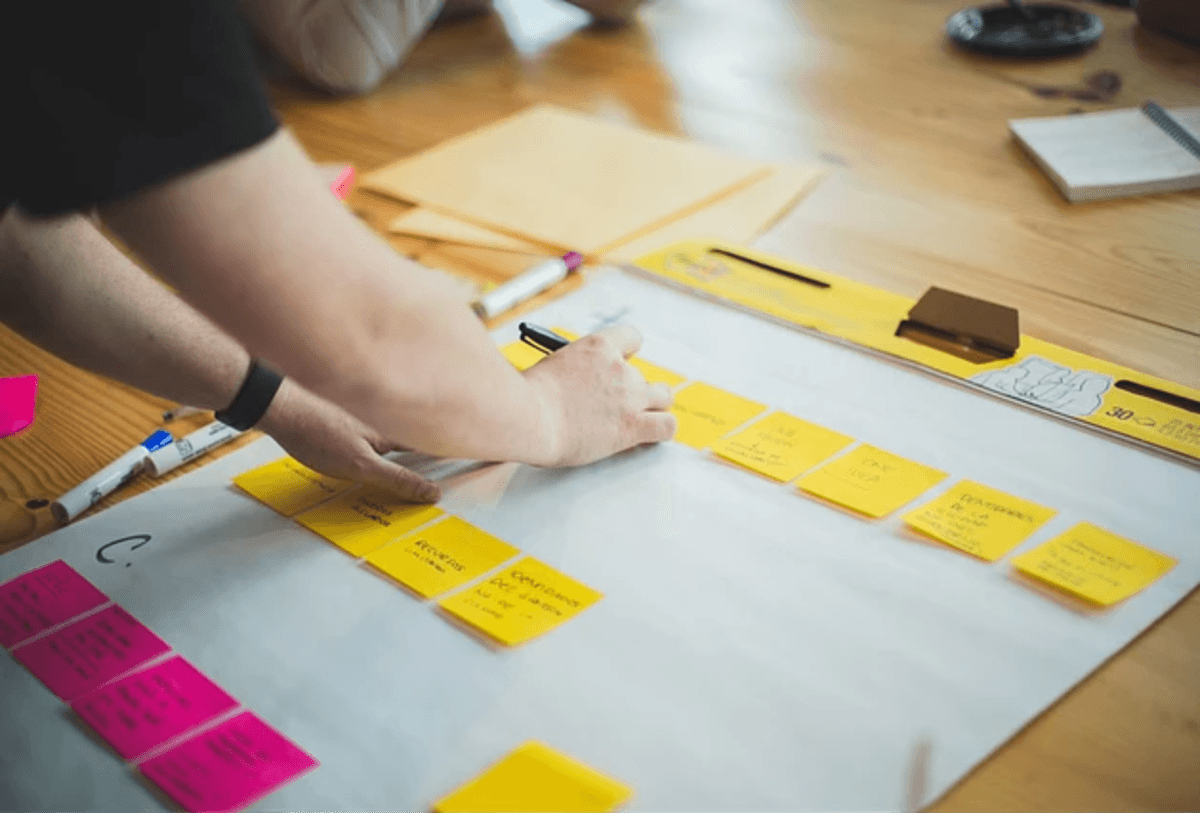
7 key areas that you need to control to effectively manage AI project lifecycle
According to recent research by RAND (published on Aug 13, 2024), over 80% of AI projects fail — which is twice the failure rate for non-AI projects. Clearly, managing machine learning projects demands a new level of vigilance.
So, what separates successful AI adoption projects from the rest? Let’s dig into the key areas you’ll need to oversee to keep your AI projects on track and delivering real value.
Cost management
As forecasted by Gartner, at least 30% of generative artificial intelligence projects will be abandoned after the proof-of-concept stage by the end of 2025, primarily due to difficulties in proving and realizing value amidst high costs. In the press release from July this year, Rita Sallam, the VP Analyst at Gartner, commented:
"After last year’s hype, executives are impatient to see returns on GenAI investments, yet organizations are struggling to prove and realize value. As the scope of initiatives widens, the financial burden of developing and deploying language models is increasingly felt."
The challenge here is capturing the monetary value of GenAI investments in a way that justifies their substantial costs. And we’re talking about truly significant figures:
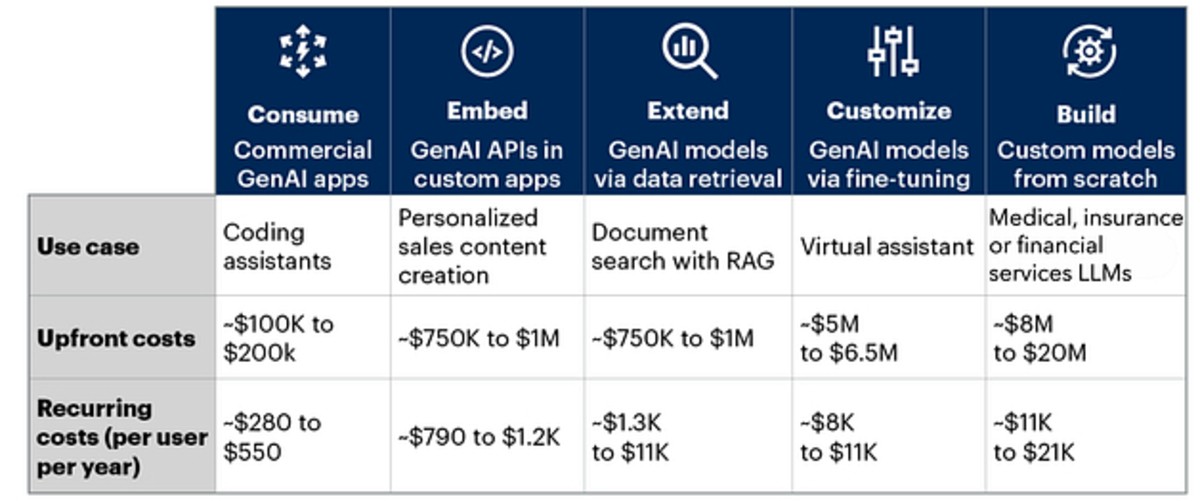
(source: Gartner, July 2024)
The key to successful implementation lies not just in adopting AI but in having a clear strategy to capture its value.
From this perspective, effective cost management is critical to any AI project success. Organizations need to carefully plan and monitor every phase of their GenAI projects to ensure they’re not only staying within budget but also generating measurable returns at each stage.
Information access
In AI projects, controlling information access is non-negotiable—both for the AI itself and for the people using it. By balancing the AI’s data needs with role-based permissions for team members, managers can build a system that supports security, compliance, and trust throughout the organization.
On one side, Gen AI systems need data to deliver insights, but giving them broad access to sensitive company information brings significant security and privacy risks. From the manager’s perspective, it’s crucial to ensure the AI only accesses the data it truly needs, with strict safeguards in place.
Equally important is managing human access to the AI’s insights and controls. In many cases, a multi-level access structure tailored to each employee’s role is essential for maintaining security and compliance – and helps to adjust AI’s insights to each team’s specific needs. By managing this area, you are creating a flexible, secure AI solution that supports diverse functions across the organization, from customer service to strategic planning.
Take an example of our recent project for a large non-profit organization. The chatbot allowed employees to retrieve information while ensuring that access levels were strictly controlled—preventing unauthorized personnel from viewing sensitive data and maintaining rigorous data compliance standards throughout the organization.
Read more: Generative-AI-powered chatbot with multi-level information access
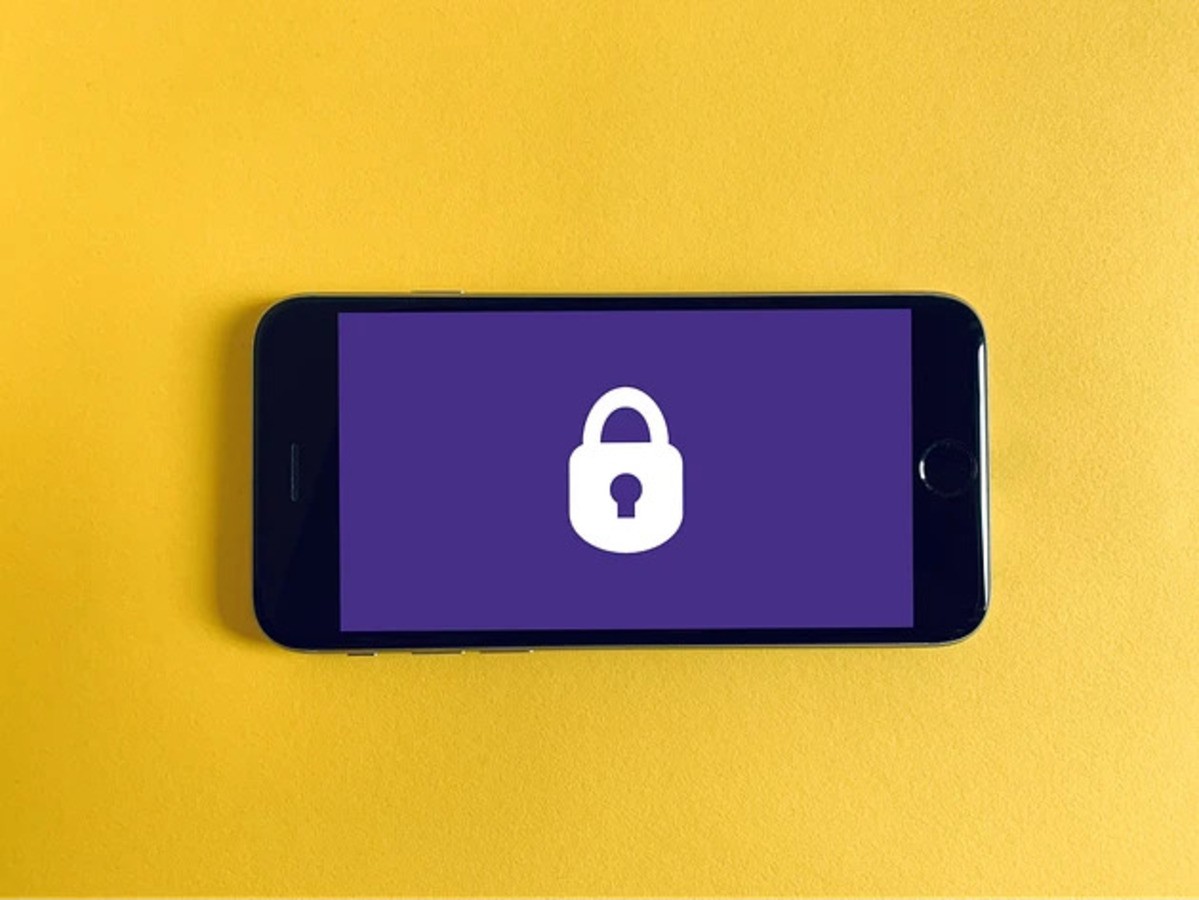
Data privacy and security
Continuing the topic of data surveillance, we can’t overlook data privacy and security. With models relying on vast amounts of sensitive information, managers must ensure data handling is airtight and fully compliant with GDPR, CCPA, and internal policies. Mishandling data, even accidentally, can lead to serious breaches, leaks, and costly regulatory penalties.
The common techniques of managing these risks include:
Encryption to protect data at rest and in transit,
Role-based access control to limit access based on need,
Regular audits to verify compliance at every stage.
Building on these essentials, there are additional layers of protection that can make a substantial difference in AI projects. First, consider implementing data minimization practices from the start. Limit the data used by the models to the absolute minimum needed for the task. This reduces exposure risk significantly and keeps compliance more manageable.
Another critical step is to embed privacy impact assessments into each stage of the project. Making privacy checks integral to each project milestone, rather than treating them as add-ons, helps catch and address vulnerabilities early on. This proactive approach reduces regulatory risks down the line and keeps all stakeholders – from the data science team to compliance officers – aligned on privacy goals at every stage.
Regulatory compliance
Beyond protecting sensitive data, AI systems – in highly regulated industries like finance, healthcare, and legal – must be designed to adhere to strict guidelines on data use, transparency, and accountability. This requires managers to incorporate compliance measures from the very start and treat them as essential to the project’s structure and long-term success.
One of the best ways to ensure compliance is to involve legal and compliance teams from the earliest stages of the project. By bringing these stakeholders in from the start, managers can embed regulatory considerations directly into the AI system’s design rather than treating compliance as an afterthought. Regular check-ins with compliance experts also help teams stay current with evolving regulations, making it easier to spot and address potential issues before they escalate.
Another essential practice to include in your AI project is a compliance audit process. It should include comprehensive model documentation, data lineage tracking, and regular validation to ensure the system adheres to current regulations—and is prepared to adapt to new ones as they emerge. As the models continuously learn and update, frequent audits are crucial to maintaining compliance as the system evolves.
Ethical considerations
As AI becomes increasingly embedded in decision-making, ethical considerations must be taken seriously to ensure that AI technology aligns with an organization’s values and social responsibilities. This means addressing biases, promoting fairness, and preventing harmful or inappropriate content generation.
To manage these risks, teams should establish robust processes for bias detection, data audits for diversity, and impact testing throughout the whole project. Safeguards, such as filtering mechanisms and human-in-the-loop oversight, help ensure that AI outputs meet organizational standards for safety and appropriateness. Regular reviews also allow teams to identify and address emerging ethical issues as the AI model evolves.
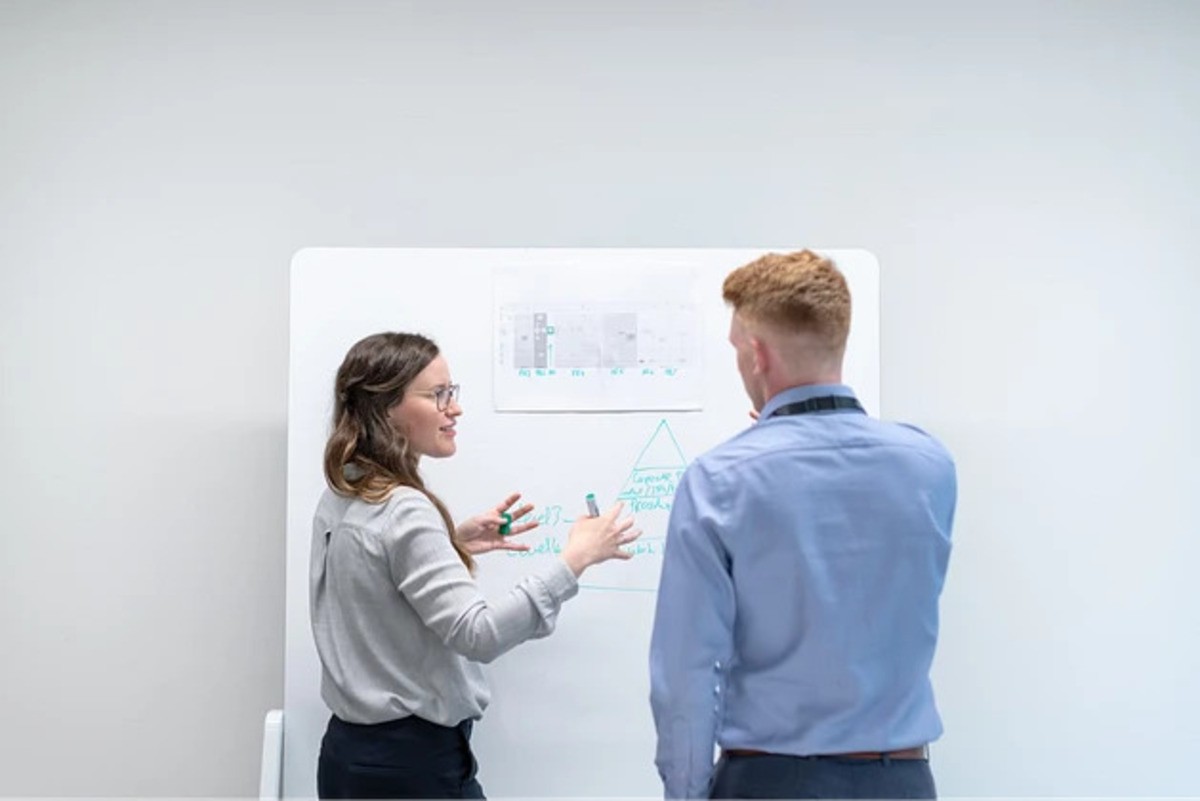
AI tools and vendor selection
Choosing the right vendors for AI projects is a strategic decision that requires careful oversight. Product owners play a key role here, balancing business needs, project requirements, and budget constraints to find solutions that truly fit. The goal is to select tools and partners that support both the project’s immediate objectives and the organization’s long-term strategy.
To evaluate tools providers and vendors effectively, consider the following:
Model Transparency: Does the vendor provide clarity on how their models function and make decisions?
Data Handling Practices: Are the vendor’s data handling and storage practices aligned with your organization’s privacy standards?
Regulatory Compliance: Does the vendor comply with industry regulations relevant to your project (e.g., GDPR, HIPAA)
Ongoing Accountability: Are there regular check-in points to assess progress, address issues, and keep the vendor aligned with evolving project needs?
Scalability and Adaptability: Can the vendor’s solution scale and adapt as your project grows or changes?
The similar points can be applied to choosing AI development services providers. A few extra hours during the assessment process can save you weeks of works and reworks.
Communication between stakeholders and AI project teams
You already know that AI projects demand constant collaboration across technical and non-technical teams – developers, product owners, compliance experts, and other stakeholders all need to work closely to keep the project aligned with business goals.
But if you’re eagerly setting up a new spreadsheet, Google Drive folder, and mailing list for endless email chains – stop right there. These fragmented approaches slow down decision-making, create unnecessary silos, and increase the risk of miscommunication.
Efficient AI projects (and, honestly, all software projects) require tools that support shared dashboards, live data integration, and direct feedback loops. These platforms make it easy for all stakeholders to track progress, share insights, and resolve issues quickly. Clear channels for regular updates and feedback ensure smooth communication so adjustments can be made promptly – keeping the project agile and focused on the end goal.
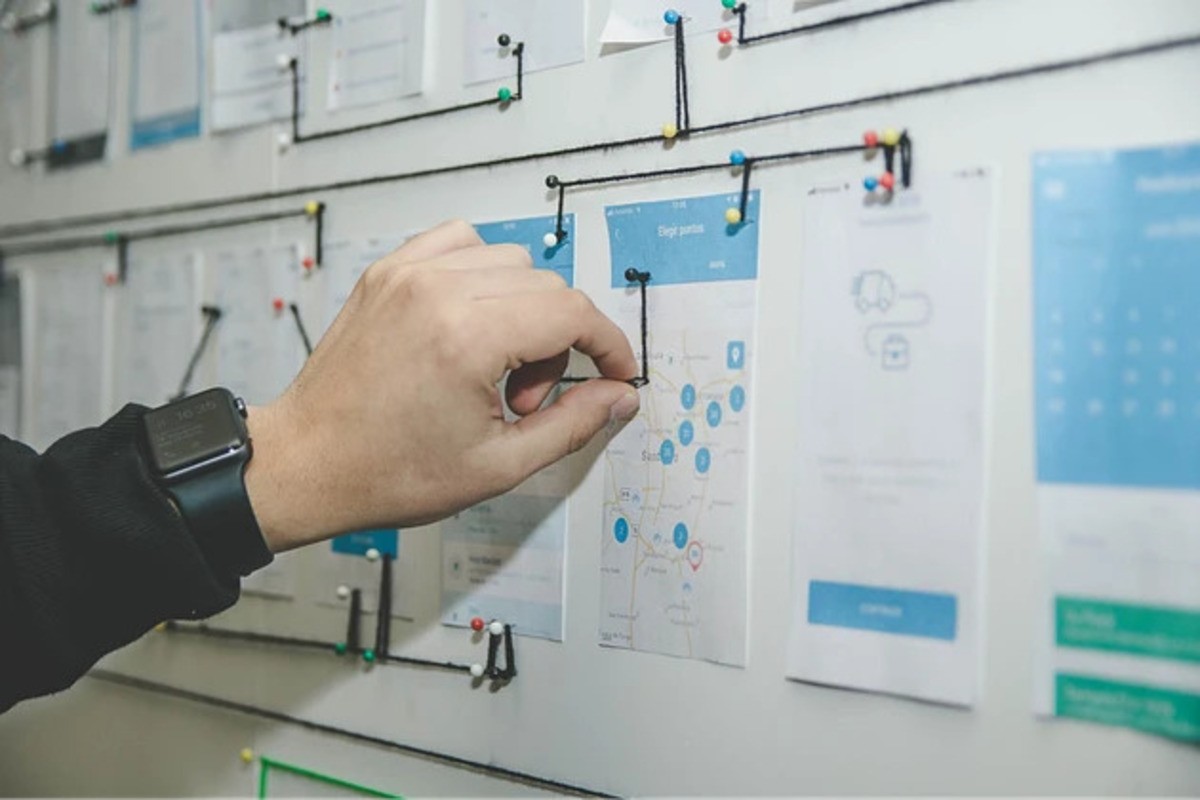
How to minimize AI project risks? Best practices of AI project development
We asked an expert in this field, Sohrab Hosseini from Orq.ai, to share his advice on minimizing the risks of AI projects.
"AI projects come with their own set of unique challenges. Next to the need to manage project costs and safeguard the privacy and security of your data, companies must equip themselves with the right tech stack to successfully navigate the challenges of building AI solutions and come out on top.
This is especially true when building solutions powered by Gen AI. This is because large language models (LLMs) generate probabilistic responses that can vary widely and sometimes “hallucinate” — producing information that appears convincing but is entirely fabricated.
To ensure these language models are accurate, reliable, and aligned with business needs, organizations need a tech stack to continuously monitor, refine, and validate AI outputs. This is where we at Orq.ai come in.
As a Generative AI Collaboration Platform for teams to build AI-powered solutions, Orq.ai delivers the tooling needed to operate large language models out of the box in a user-friendly interface and covers the workflow to take AI products from prototype to production safely.”
Now, let’s take a look at how various AI project risks can be minimized and how Orq.ai platform streamlines this process.
Human-in-the-Loop (HITL)
In AI project supervision, one of the biggest challenges is establishing smooth, ongoing communication between software engineers, product teams, and subject-matter experts to continuously refine and improve AI outputs. A HITL approach is essential for successful Gen AI projects because it integrates human judgment into AI model finetuning and error correction, thus preventing models from operating in isolation and ensuring they stay aligned with real-world requirements and expectations.
At Orq.ai, we address this challenge by enabling HITL capabilities within our platform to allow any team member to participate in the AI improvement process, whether they have a technical or non-technical background. Through our Generative AI Collaboration Platform, users can log feedback on AI outputs, flag inaccuracies, and make correction suggestions in one place. This feedback can be submitted directly through Orq.ai’s user interface or programmatically via an API, accommodating diverse team workflows. Each piece of feedback can then be transformed into actionable data, contributing to a dataset that drives continuous model improvement.
Overview of “feedback” section in Orq.ai’s platform allowing users to provide or annotate feedback on an AI-generated response.
By infusing this collaborative HITL framework within our platform, Orq.ai empowers teams to streamline communication across all contributors, fostering more accurate, relevant output in a simple, integrated process.
Cost Management
Managing costs effectively is critical in Gen AI projects. Each interaction with a large language model, be it during development, testing, or production, incurs a cost that is typically influenced by model complexity, data volume, and processing power required. These costs can escalate quickly in cases where LLMs are frequently queried. Without visibility into these costs, teams risk exceeding budgets or underutilizing resources, making cost control an essential component of AI project management.
Orq.ai’s platform addresses this challenge by providing real-time insights into model interactions and associated costs. With the Logs Overview feature, teams can track each interaction with LLMs, viewing detailed information such as the model provider, execution time, and costs incurred per call. This transparency equips teams to monitor expenses closely, enabling them to make informed decisions about resource allocation and usage patterns.
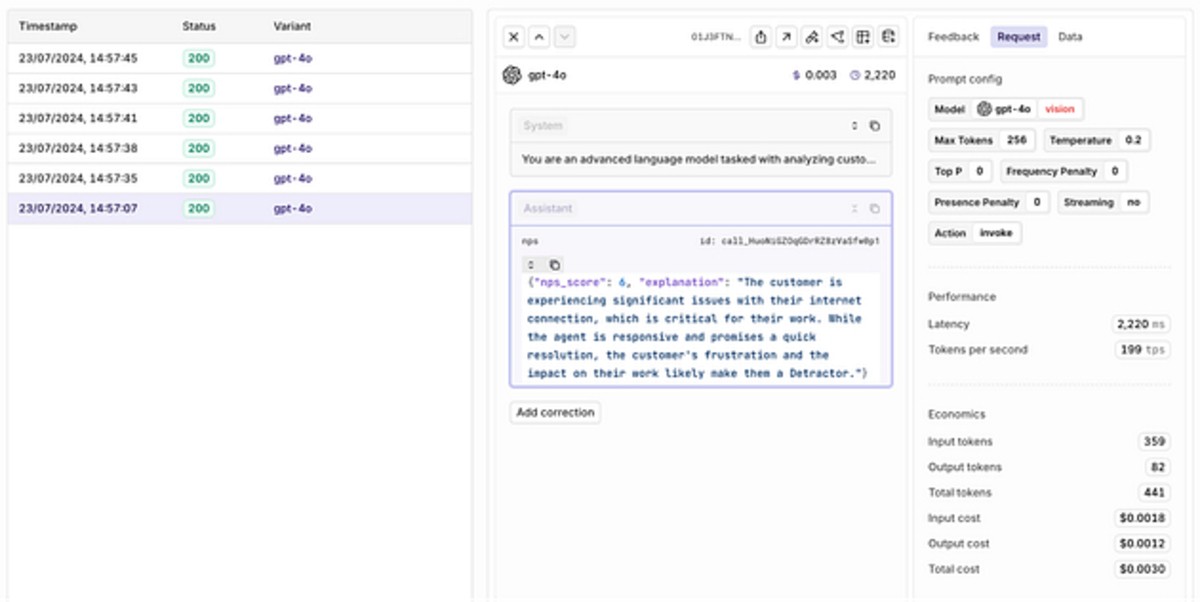
In Orq.ai’s “Requests” Panel, users can see all model configurations as well as the execution latency and cost.
With this detailed cost data, teams can better manage their budgets, making adjustments to model configurations or switching providers if needed to achieve an optimal balance of performance and cost efficiency.
Data Privacy & Security
In AI project management, working with data-enriched models demands that teams safeguard sensitive information to maintain data privacy. Orq.ai’s platform addresses these needs by integrating privacy and security measures directly into the AI development workflow, helping organizations confidently manage sensitive data.
SOC2 Certification and Private Cloud Deployments
To ensure rigorous security, Orq.ai is SOC2 certified, adhering to high data protection and privacy standards. This certification provides a baseline for secure data handling, which is especially important in regulated industries. Orq.ai can also be deployed within private cloud environments on platforms like Azure, AWS, and Google Cloud Platform. By operating within an organization’s isolated virtual network, this setup minimizes data exposure risks and adds an essential layer of control over data storage and processing.
Personal Information Protection
Orq.ai supports granular data privacy controls by allowing teams to identify and protect Personally Identifiable Information (PII). For AI projects involving sensitive information, users can flag PII within datasets, ensuring it’s automatically censored from logs and not stored in Orq.ai. This functionality allows teams to work with essential AI features while preventing sensitive information from being visible or stored unnecessarily.
Output and Input Masking
To further enhance data security, Orq.ai includes input and output masking options. This allows organizations to conceal sensitive information in AI model input and output, ensuring that private data is never stored within Orq.ai’s system.
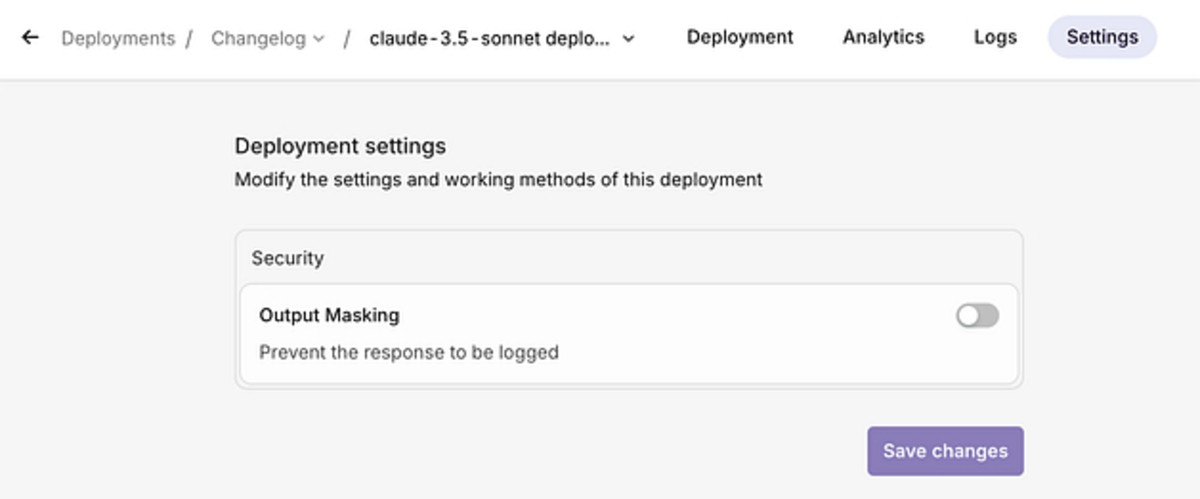
This feature is particularly valuable for use cases like customer support chatbots or financial advisory tools, where model responses might inadvertently reveal personal or confidential data.
By implementing these privacy-focused features, Orq.ai provides teams with tools to control data security and privacy while managing AI projects.
Successful AI project management – key takeaways
When it comes to AI projects, success isn’t just about building cutting-edge models—it’s about creating solutions that drive real business impact. Leveraging AI capabilities requires you to keep up with the latest advancements while maintaining a clear focus on strategy and execution throughout the project: from exploring AI initiatives and implementing solutions to optimizing existing tools for better performance.
The success of an AI project depends on striking the right balance between innovation and oversight. By addressing key challenges like cost management, data privacy, and stakeholder collaboration, you can ensure your AI initiatives stay aligned with your goals while delivering measurable results.
Good luck with your next project! And if you need a hand, we are always ready to help.