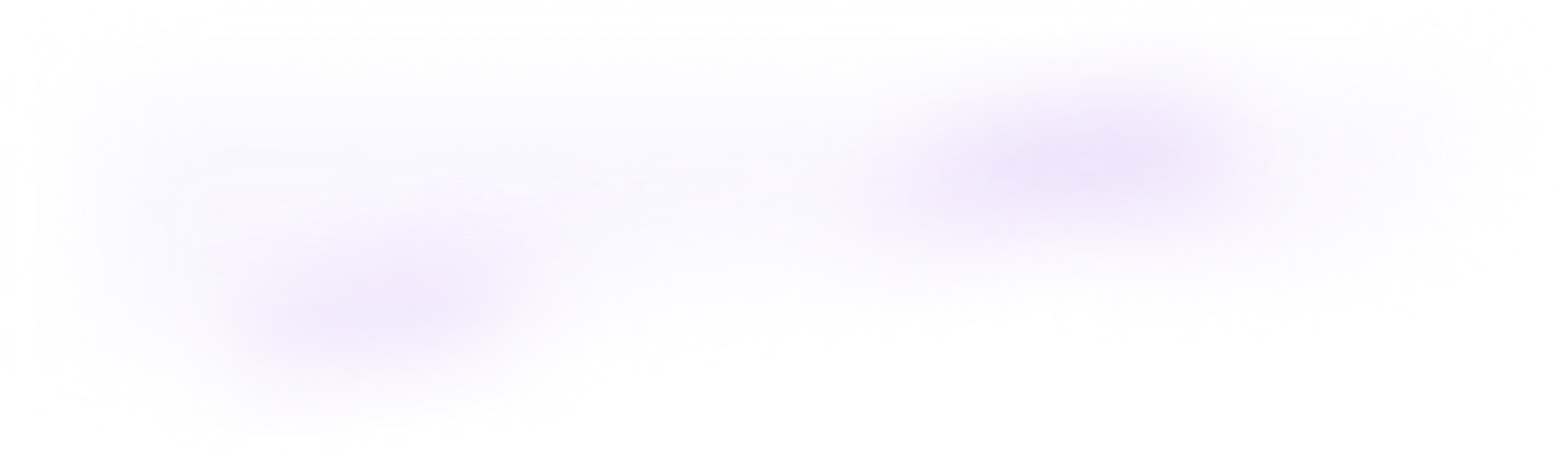
Top 6 LangSmith Alternatives in 2025: A Complete Guide
Explore top LangSmith alternatives, including Orq.ai, to find the ideal platform for optimizing, deploying, and monitoring your LLM applications.
January 9, 2025
Author(s)
Key Takeaways
LangSmith alternatives offer diverse solutions, from open-source frameworks to comprehensive observability platforms.
Each tool is tailored to specific needs, such as model explainability, user engagement, or cost-effective scalability.
Orq.ai stands out as an all-in-one platform for developing, deploying, and optimizing LLM applications.
LangSmith is an advanced tool designed for LLM observability, helping developers monitor and improve the performance of language models (LLMs). This platform offers features like tracing, prompt versioning, and experimentation, enabling teams to fine-tune their AI models for optimal performance. As the demand for LLM applications continues to grow, LangSmith’s robust suite has made it a go-to for LLM monitoring.
However, some users might seek LangSmith alternatives for various reasons, such as cost analysis, specific self-hosted deployment needs, or the desire for a more flexible pricing model. With the rapid evolution of LLM observability tools, there are now multiple solutions on the market that provide similar capabilities, often with unique features or better pricing structures.
In this guide, we’ll explore the top 6 LangSmith alternatives in 2025, offering insights into their key features, pros, and how they compare in terms of evaluation metrics, performance, and flexible pricing. If you're considering a switch from LangSmith or exploring other options for your LLM needs, read on to discover the best alternatives available.
Criteria for Evaluating LLM Observability Tools
When selecting the best LangSmith alternatives, it's essential to evaluate the tools based on several key criteria that align with your organization's needs and technical requirements. The right observability tool should offer comprehensive features that support both LLM performance monitoring and optimization.
1. Open-Source Availability and Alternatives
For teams that prefer to modify and customize their observability tools, choosing an open-source alternative is often a priority. Open-source platforms offer transparency, flexibility, and the ability to tailor the tool to specific needs. These platforms also benefit from community contributions, ensuring they evolve with industry trends. However, it’s worth noting that there are also excellent non-open-source alternatives that provide powerful features and flexibility without the need for code customization. These tools often offer robust support and maintenance, along with additional enterprise-grade features that can be valuable for teams looking for comprehensive solutions with minimal setup and maintenance requirements.
2. Self-Hosting Capabilities
A key consideration for many organizations is the ability to self-host the observability tool. This option allows for greater control over data, security, and the customization of monitoring systems. Whether you are managing sensitive information or simply prefer on-premise solutions, self-hosting can offer peace of mind and enhanced flexibility.
3. Support for Prompt Templating and Agent Tracing
Effective prompt templating is crucial for maintaining consistency and streamlining workflows. Many LLM observability tools provide the ability to define reusable prompts that can be customized for different use cases. Agent tracing also plays an important role in understanding how an agent's behavior evolves during interactions with language models, helping developers track performance issues and refine agent design.
4. Experimentation and Evaluation Features
Experimentation is vital in optimizing language models. Tools that support experiments and allow for the creation of prompt experiments provide a structured way to test different configurations and compare model outputs. Features such as traces visualization make it easier to analyze and debug these experiments by giving developers clear insights into the flow of interactions and model outputs.
5. Cost Analysis Tools
Given that LangSmith pricing can be a concern for many users, tools that provide built-in cost analysis features are essential. These tools help track resource consumption, assess usage patterns, and predict future costs based on model utilization. Understanding the financial impact of observability tools allows organizations to make data-driven decisions about scalability and budget allocation.
6. User and Feedback Tracking
Incorporating user tracking is another critical factor in evaluating observability tools. By collecting feedback and tracking user interactions with the language model, these tools enable teams to understand how models are performing in real-world scenarios. Feedback can be used to refine models and improve performance over time. Additionally, tools that support user tracking often include customizable custom properties for segmenting users based on behavior or other attributes.
7. Integration with Frameworks Like LangChain
Seamless LangChain integration and compatibility with other machine learning frameworks are essential for maximizing the utility of your observability tool. A tool that can easily integrate with existing infrastructure and other tools used in the machine learning lifecycle will save time and enhance collaboration. Consider whether the tool can be incorporated into your existing machine learning observability stack without extensive customization.
8. Pricing Flexibility
Pricing flexibility is another vital consideration when choosing an observability tool. Whether you're looking for an open-source alternative or a commercial solution with various pricing tiers, it's important to assess how pricing structures align with your team's budget. Flexible pricing can make a significant difference for startups, small teams, and enterprises alike, as it provides scalability as usage increases.
9. Support for Various Data Types (e.g., Text, Images)
LLM applications are not limited to text-based models. Observability tools that support a variety of datasets, such as images, audio, and text, offer the flexibility to monitor and analyze multimodal AI models. This makes them highly valuable for teams working on diverse AI projects that go beyond traditional text generation and require the handling of different types of data.
10. Dashboard and Data Export Functionalities
Finally, a comprehensive dashboard is essential for visualizing model performance, tracking metrics, and monitoring the progress of experiments. Effective dashboards provide both high-level overviews and granular insights into performance. Additionally, the ability to export data in different formats, such as CSV or JSON, is crucial for analyzing performance offline or integrating with other systems for advanced analytics.
6 Best Alternatives to LangSmith
1. Orq.ai
Orq.ai is an advanced Generative AI Collaboration Platform designed to help AI teams develop, deploy, and optimize large language models (LLMs) at scale. Launched in February 2024, Orq.ai provides a powerful suite of tools that streamline the entire AI application lifecycle. With its integration capabilities and user-friendly interface, Orq.ai is transforming how teams interact with AI models and deploy them to production.
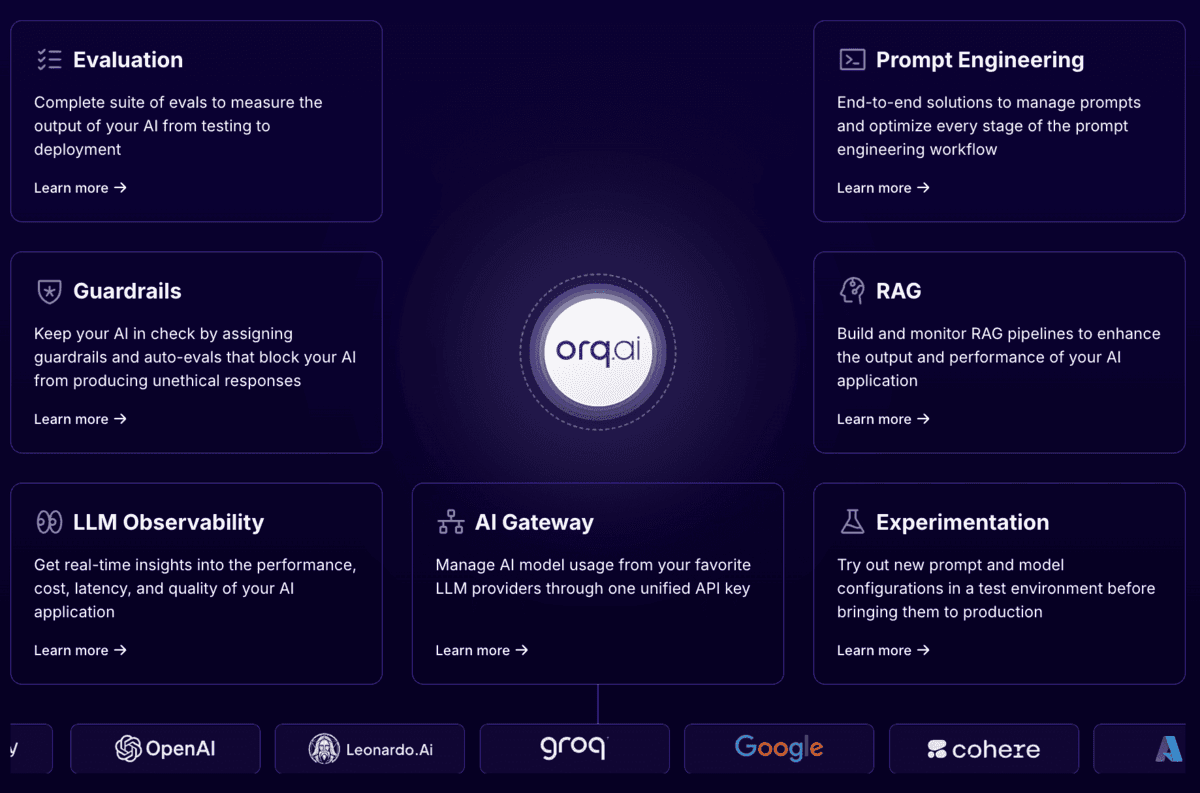
Orq.ai Platform Overview
Key Features:
Generative AI Gateway: Orq.ai integrates seamlessly with 130+ AI models from leading LLM providers. This flexibility allows organizations to experiment with different models and select the best fit for their use cases, making it a strong contender among LangChain alternatives.
Playgrounds & Experiments: Teams can run controlled sessions to test and compare AI models, prompt configurations, and Retrieval-Augmented Generation (RAG)-as-a-Service pipelines. This experimentation environment empowers AI teams to hypothesize and evaluate AI behaviors, improving their performance optimization processes before moving to production.
AI Deployments: With built-in guardrails, fallback models, and regression testing, Orq.ai ensures dependable AI deployments. Teams can monitor AI models in real time, reducing the risks associated with moving AI applications from staging to production.
Observability & Evaluation: Orq.ai provides detailed monitoring and intuitive dashboards for real-time performance tracking. By integrating programmatic, human, and custom evaluations, teams can continuously measure and optimize performance. The platform’s model drift detection tools help identify and correct changes in model behavior, ensuring sustained accuracy over time.
Security & Privacy: With SOC2 certification and compliance with GDPR and the EU AI Act, Orq.ai meets stringent data security and privacy requirements, providing peace of mind for organizations handling sensitive information.
Why Choose Orq.ai?
Orq.ai offers a comprehensive, end-to-end solution for managing LLM observability, from initial development through to deployment and ongoing optimization. Whether you're looking for LangChain alternatives or a platform that supports model drift detection and annotation for detailed analysis, Orq.ai delivers the tools necessary for scaling and optimizing AI applications efficiently. It bridges the gap between technical and non-technical teams, making it easy for everyone to collaborate on AI projects and deploy at scale.
However, as a relatively new player in the market, Orq.ai may have fewer community-driven resources and third-party integrations compared to more established platforms. Teams accustomed to longer-term, highly-supported tools might need to invest time in exploring and adopting the platform fully.
To learn more about how Orq.ai can help streamline your AI workflows, book a demo today.
2. Helicone
Helicone is an open-source framework for LLM observability and monitoring, specifically designed for developers who need to efficiently track, debug, and optimize large language models. Offering both self-hosted and gateway deployment options, Helicone provides the flexibility to scale observability efforts without sacrificing control or performance. It is a strong competitor among LangChain competitors and is well-suited for teams that prefer open-source solutions with the ability to customize the platform according to their needs.

Credits: helicone.ai
Key Features:
Sessions for Tracking Multi-Step Agent Workflows: Helicone allows developers to track and visualize multi-step workflows across different agents, making it easier to monitor performance and troubleshoot issues as they arise. This debugging capability is critical for teams working with complex LLM applications that require detailed workflow tracking.
Prompt Versioning and Experimentation: Helicone supports prompt versioning, allowing teams to test and compare different prompt configurations. This makes it easier to experiment with various versions of prompts and assess their impact on model behavior, ensuring optimal performance over time.
Custom Properties for User Segmentation: Helicone enables teams to segment users based on custom properties, providing the ability to track performance and gather insights from different user groups. This level of granularity helps improve LLM observability and ensures that model behavior is understood in context.
Self-Hosted or Gateway Options: For teams looking for self-hosted solutions, Helicone offers the flexibility to run the platform on-premise, giving complete control over the deployment environment. Alternatively, teams can use the gateway option for a quicker, cloud-based deployment without compromising scalability.
Support for Text and Image Inputs/Outputs: Helicone supports both text and image inputs/outputs, enabling teams to monitor and optimize multimodal LLM applications. This flexibility makes Helicone an excellent choice for teams working with diverse data types beyond text.
Volumetric Pricing Model: Helicone offers a flexible, volumetric pricing model based on usage, which is ideal for teams looking for cost-effective scalability. This model ensures that organizations only pay for what they use, making Helicone a budget-friendly option for both small teams and larger enterprises.
Why Choose Helicone?
Helicone is a strong LLM observability alternative, especially for developers seeking an open-source platform that offers flexibility and scalability. While it excels in providing cost-effective and customizable solutions for developers, it may not be the best fit for larger enterprises or non-technical teams due to its limited enterprise features and steeper learning curve.
Phoenix Arize AI
Phoenix by Arize AI is a specialized platform designed to help teams monitor, evaluate, and optimize their AI models at scale. With its focus on model explainability, Phoenix provides advanced tools for tracking and improving the performance of large-scale AI systems. For organizations seeking to dive deeper into evaluation metrics and detect model drift, Phoenix offers a robust solution.

Credits: Phoenix Arize AI
Key Features:
Advanced Evaluation Metrics and Performance Optimization: Phoenix provides granular insights into model performance, allowing teams to track specific metrics and fine-tune their AI systems for peak efficiency. Its tools for performance optimization are particularly valuable for long-term system improvement.
Drift Detection and Model Explainability Tools: Phoenix excels at model drift detection, identifying when models start to deviate from expected behaviors due to changes in data patterns. These insights, coupled with explainability features, help teams maintain trust and transparency in their AI systems.
Flexible Pricing Model: Phoenix offers pricing plans tailored to startups and enterprises, ensuring accessibility for organizations of various sizes. This makes it a viable option whether you're an emerging business or a large-scale enterprise.
Why Choose Phoenix?
Phoenix stands out for its emphasis on deep insights into the performance and behavior of LLM applications. Its comprehensive tools for tracking model drift and providing actionable explanations make it an excellent choice for teams working with high-stakes AI models.
However, while Phoenix shines in explainability and evaluation, its narrower focus may not cater to teams looking for an all-in-one solution. For organizations seeking a broader set of observability features—such as prompt templating or full deployment capabilities—it might be necessary to pair Phoenix with other tools.
4. Langfuse
For teams seeking an open-source alternative to LangSmith, Langfuse delivers a powerful and transparent platform for LLM observability. Its self-hosted architecture ensures that teams maintain full control over their data and deployment environments, making it an attractive option for organizations prioritizing customization and data security.

Credits: Langfuse
Key Features:
Full Open-Source Platform with Self-Hosted Options: Langfuse’s commitment to open-source ensures that teams can modify and adapt the platform to meet their specific needs. The option to self-host adds another layer of flexibility for organizations that require greater control over their observability workflows.
Real-Time Tracing and Prompt Templating: With support for real-time tracing, Langfuse enables teams to monitor LLM interactions and troubleshoot effectively. Its prompt templating tools further streamline the process of creating, testing, and optimizing prompts for better performance.
Community-Driven Development with Extensive Documentation: Backed by a vibrant open-source community, Langfuse evolves rapidly based on user feedback. The platform also provides detailed documentation, making it easier for teams to onboard and implement the tool effectively.
Why Choose Langfuse?
Langfuse is an ideal solution for teams that prioritize transparency, customization, and the ability to self-host their observability platform. Its open-source nature means that organizations can adapt the tool as their needs evolve, while the community-driven development ensures continuous updates and improvements.
However, teams considering Langfuse should be aware that relying on an open-source framework might require more internal resources for setup, maintenance, and scaling. For organizations without dedicated technical expertise, the self-hosted deployment option could introduce additional complexity.
5. HoneyHive
HoneyHive distinguishes itself as a LangSmith alternative by emphasizing user tracking and engagement analytics, making it a strong choice for teams that prioritize customer experience and application optimization. Designed with startups and smaller companies in mind, HoneyHive’s affordability and intuitive interface enable teams to gather actionable insights without overextending their budgets.`

Credits: Honeyhive
Key Features:
Focus on User Tracking and Engagement Analytics: HoneyHive provides tools to monitor how users interact with your AI applications, enabling teams to track behaviors, gather feedback, and identify areas for improvement. This level of insight is invaluable for enhancing the overall user experience.
Customizable Dashboard and Feedback Tracking Tools: Teams can leverage a customizable dashboard to visualize metrics that matter most. Paired with robust feedback tracking tools, HoneyHive ensures that user insights are captured and acted upon effectively.
Affordable Cost Analysis Features: HoneyHive offers built-in cost analysis capabilities, helping teams evaluate the performance of their LLM applications against budget constraints. This feature is especially helpful for startups and smaller organizations looking to optimize costs while maintaining performance.
Why Choose HoneyHive?
HoneyHive is a standout solution for teams aiming to merge LLM observability with a focus on user feedback. Its combination of affordable pricing, intuitive analytics, and robust engagement tools makes it a compelling option for those prioritizing customer experience and performance optimization.
However, while HoneyHive excels in user engagement and feedback tracking, its feature set may not be as comprehensive as some other platforms in terms of advanced evaluation or model drift detection. Larger enterprises or teams needing deeper AI optimization features might find it more suitable as a supplementary tool rather than a standalone solution.
6. OpenLLMetry by Traceloop
OpenLLMetry, developed by Traceloop, is an open-source observability tool specifically designed for LLM applications. It provides developers with powerful tools for tracking and optimizing LLM performance while offering the flexibility to customize and adapt the platform to fit specific workflows.

Credits: Traceloop
Key Features:
Agent Tracing: OpenLLMetry enables detailed agent tracing, allowing developers to monitor and debug multi-step workflows within their LLM applications. This capability ensures greater transparency and efficiency in troubleshooting.
Prompt Templating: With support for prompt templating, OpenLLMetry streamlines the process of creating, testing, and optimizing prompts. Developers can iterate quickly to improve performance and reliability.
Experimentation Features: OpenLLMetry includes tools for running experiments, making it easy to test different configurations and evaluate the impact of changes on model behavior. This experimentation capability is critical for teams focused on continuous improvement.
Why Choose OpenLLMetry?
OpenLLMetry is best suited for developers looking for a community-driven, customizable solution for LLM observability. Its open-source nature and focus on transparency make it a reliable choice for teams wanting to tailor their observability tools to their unique needs.
However, it’s worth noting that as a community-supported platform, OpenLLMetry may lack the dedicated customer support or polished user experience of proprietary tools. Teams without sufficient technical expertise or resources might find setup and maintenance more challenging compared to commercial options.
Langsmith Alternatives: Key Takeaways
Selecting the best alternative to LangSmith depends on your team’s unique requirements, whether it’s the flexibility of an open-source framework, advanced tools for model explainability, or robust user engagement analytics. Each platform we’ve discussed—Helicone, Phoenix by Arize AI, Langfuse, HoneyHive, and OpenLLMetry—brings something valuable to the table, offering diverse solutions tailored to different use cases and priorities.
For teams seeking an end-to-end LLMOps platform, Orq.ai stands out as a versatile and user-friendly solution. By combining features like seamless integration capabilities, real-time performance optimization, and advanced evaluation metrics, Orq.ai bridges the gap between technical and non-technical stakeholders, empowering teams to develop, deploy, and optimize LLM applications at scale.
As the landscape of LLM observability continues to evolve, choosing a platform that aligns with your goals is essential. Whether you prioritize customization, scalability, or a comprehensive toolset, the right choice can significantly impact your AI application’s success.
Ready to see how Orq.ai can revolutionize your AI workflows? Book a demo today and explore the possibilities.